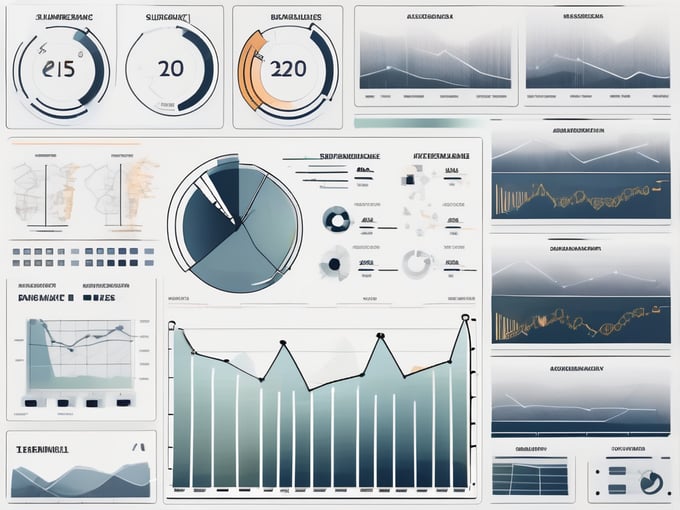
Important Trends in Data Analytics for Asset Management Specialists in the Sludge Management Industry
In today's rapidly evolving technological landscape, data analytics has become an indispensable tool for asset management specialists in the sludge management industry. The ability to harness and analyze vast amounts of data can provide invaluable insights, optimize operations, and drive efficient decision-making processes. This ultimate guide aims to explore the importance of data analytics in sludge management, delve into fundamental concepts, discuss relevant tools, provide guidance on implementing data analytics, highlight future trends, and conclude with key takeaways. So, let's dive in!
Understanding the Importance of Data Analytics in Sludge Management
The role of data analytics in asset management cannot be understated. In the context of sludge management, it enables specialists to gain a comprehensive understanding of their assets, assess their performance, and make informed decisions to improve efficiency and mitigate risks.
Sludge management is a critical aspect of wastewater treatment plants and other industrial processes. It involves the proper handling, treatment, and disposal of the residual solids that accumulate during the treatment process. Effective sludge management is essential to ensure compliance with environmental regulations, minimize the impact on public health, and optimize the overall operation of the facility.
The Role of Data Analytics in Asset Management
Data analytics plays a crucial role in asset management by providing insights into asset condition, performance, and lifecycle. By analyzing data collected from sensors, monitoring systems, and maintenance records, specialists can identify potential issues, predict failures, and optimize maintenance strategies.
When it comes to sludge management, data analytics can help monitor the performance of various assets involved in the process. This includes pumps, mixers, dewatering equipment, and sludge treatment systems. By continuously collecting and analyzing data from these assets, specialists can gain valuable insights into their operational efficiency, energy consumption, and maintenance requirements.
Furthermore, data analytics can also assist in identifying patterns and trends in sludge characteristics, such as its composition, volume, and consistency. This information can be used to optimize the treatment process, adjust chemical dosing, and improve overall sludge quality.
Key Benefits of Implementing Data Analytics in Sludge Management
Integrating data analytics into sludge management practices offers numerous benefits. Firstly, it allows for real-time monitoring, enabling quick detection of anomalies and abnormalities in sludge processing and treatment. This early detection can help prevent equipment failures, minimize downtime, and reduce the risk of environmental contamination.
Secondly, data analytics facilitates evidence-based decision-making by providing accurate and timely information. By analyzing historical data and real-time performance metrics, specialists can make informed decisions regarding maintenance activities, process optimization, and resource allocation. This data-driven approach can lead to cost savings, improved operational efficiency, and enhanced regulatory compliance.
Lastly, data analytics enhances asset performance by optimizing maintenance schedules, reducing downtime, and maximizing resource utilization. By analyzing asset performance data, specialists can identify opportunities for preventive maintenance, implement condition-based monitoring strategies, and prioritize maintenance activities based on asset criticality. This proactive approach can extend asset lifespan, improve reliability, and minimize the risk of unexpected failures.
In conclusion, data analytics plays a crucial role in sludge management by providing valuable insights into asset performance, process optimization, and decision-making. By harnessing the power of data, specialists can improve efficiency, reduce risks, and ensure the effective management of sludge in wastewater treatment plants and other industrial facilities.
Fundamental Concepts of Data Analytics
Before delving into the intricacies of data analytics, it is essential to grasp the fundamental concepts that underpin this field. Understanding these concepts will provide a solid foundation for navigating the complex world of data analysis.
Data analytics involves the extraction, transformation, and analysis of data to uncover meaningful patterns, trends, and insights. It is a multidisciplinary field that combines knowledge from statistics, mathematics, computer science, and domain expertise. By harnessing the power of data, organizations can make informed decisions, optimize processes, and gain a competitive edge in today's data-driven world.
Introduction to Data Analytics
Data analytics is a broad term that encompasses various techniques and methodologies. At its core, it revolves around the exploration and interpretation of data to extract valuable information. This information can be used to answer questions, solve problems, and make predictions.
Statistical analysis is one of the key techniques used in data analytics. It involves applying mathematical models and algorithms to analyze data and draw conclusions. By examining the distribution, variability, and relationships within the data, statisticians can uncover insights and make inferences about the population from which the data was collected.
Machine learning is another powerful tool in the data analytics toolbox. It involves training algorithms to learn from data and make predictions or take actions without being explicitly programmed. Machine learning algorithms can identify patterns, classify data, and make recommendations based on historical data.
Predictive modeling is a subset of machine learning that focuses on using historical data to make predictions about future events or outcomes. By building models based on historical patterns and trends, data analysts can forecast future behavior and make informed decisions.
Data Collection and Processing
Collecting and processing data is a crucial step in data analytics. Without high-quality data, the analysis will be flawed and the insights derived will be unreliable. Data can be collected from various sources, including databases, spreadsheets, web scraping, and sensors.
Ensuring data quality is of utmost importance. Data analysts need to verify the accuracy, completeness, and consistency of the data before proceeding with the analysis. This may involve cleaning the data, removing duplicates, handling missing values, and addressing outliers.
Organizing the data in a format suitable for analysis is also essential. This may involve transforming the data into a structured format, such as a relational database, or preparing it for analysis using specialized tools and software. Modern technologies, such as Internet of Things (IoT) sensors and automation systems, have simplified the data collection process by automatically collecting and transmitting data in real-time.
Data Analysis and Interpretation
Once the data is collected and processed, it is time to analyze and interpret the findings. Data analysis involves applying various statistical techniques, data visualization tools, and machine learning algorithms to extract meaningful insights from the data.
Statistical techniques, such as hypothesis testing, regression analysis, and clustering, are used to uncover patterns, correlations, and trends within the data. These techniques help data analysts understand the relationships between variables, identify outliers, and make predictions.
Data visualization plays a crucial role in data analysis. By representing data visually through charts, graphs, and interactive dashboards, analysts can communicate complex information in a clear and concise manner. Data visualization tools, such as Tableau and Power BI, enable users to explore data, discover patterns, and gain insights at a glance.
Machine learning algorithms are also employed to analyze data and make predictions. These algorithms can automatically learn from historical data and make accurate predictions or classifications. For example, in the sludge management industry, machine learning algorithms can be used to predict the optimal time for sludge removal based on historical data on sludge accumulation rates, weather conditions, and operational parameters.
Interpreting the results of data analysis requires domain expertise and a deep understanding of the industry or problem at hand. Data analysts need to be able to translate the findings into actionable insights and recommendations that can drive business decisions and improve processes.
In conclusion, data analytics is a multidisciplinary field that involves the extraction, transformation, and analysis of data to uncover meaningful patterns, trends, and insights. By understanding the fundamental concepts of data analytics, organizations can harness the power of data to make informed decisions and gain a competitive edge in today's data-driven world.
Data Analytics Tools for Asset Management
In the realm of data analytics, a wide range of tools and technologies are available to aid asset management specialists in their endeavors. These tools not only enhance the efficiency of data analysis but also provide valuable insights that can drive informed decision-making.
When it comes to data analytics tools, there is no shortage of options. From open-source solutions to commercial software, asset management professionals have a plethora of choices at their disposal. These tools offer various functionalities and capabilities, allowing users to extract meaningful information from vast amounts of data.
Overview of Data Analytics Tools
Let's take a closer look at some of the most commonly used data analytics tools in the asset management industry:
- Python: Known for its versatility and extensive libraries, Python is a popular choice among data analysts. Its ability to handle large datasets and perform complex calculations makes it an invaluable tool for asset management specialists.
- R: Another widely used programming language, R is particularly favored for its statistical analysis capabilities. With its vast collection of packages and functions, R enables asset management professionals to explore data, build models, and visualize results.
- SQL: Structured Query Language (SQL) is essential for managing and manipulating databases. Asset management specialists often use SQL to extract and transform data, allowing for efficient analysis and reporting.
- Tableau: Tableau is a powerful data visualization tool that enables users to create interactive dashboards and reports. With its user-friendly interface and drag-and-drop functionality, asset management professionals can easily explore data and communicate insights effectively.
- Power BI: Developed by Microsoft, Power BI is another popular choice for data analytics. It offers a wide range of data connectors, interactive visualizations, and collaboration features, making it a comprehensive tool for asset management specialists.
Each of these tools possesses its own strengths and features, catering to different data analysis requirements. Asset management professionals often leverage a combination of these tools to maximize their analytical capabilities and gain a holistic view of their assets.
Selecting the Right Data Analytics Tool
Choosing the right data analytics tool is essential to ensure optimal results in asset management. With the abundance of options available, it is crucial to consider various factors before making a decision.
First and foremost, asset management professionals need to assess the complexity of their data. Some tools may be better suited for handling large datasets or performing advanced statistical analysis, while others may excel in data visualization and reporting.
Scalability is another critical factor to consider. As asset management operations grow, the chosen tool should be able to accommodate increasing data volumes and handle complex calculations efficiently.
Usability is also a key consideration. The tool should have an intuitive interface and user-friendly features that enable asset management professionals to navigate and manipulate data effectively.
Compatibility with existing systems is yet another important aspect to evaluate. The chosen tool should seamlessly integrate with other software and databases used in the asset management process, ensuring smooth data flow and minimizing disruptions.
Conducting a thorough evaluation and understanding the specific needs of the asset management industry will aid in selecting the most suitable data analytics tool. By choosing the right tool, asset management professionals can unlock the full potential of their data and make data-driven decisions that optimize asset performance and drive business growth.
Implementing Data Analytics in Sludge Management
Embarking on the journey of implementing data analytics in sludge management can be challenging but highly rewarding. By following a strategic approach, specialists can overcome obstacles and reap the benefits of data-driven decision-making.
Sludge management is a critical aspect of wastewater treatment plants. It involves the proper handling, treatment, and disposal of the residual sludge generated during the treatment process. Traditionally, sludge management has relied on manual processes and subjective decision-making. However, with the advancements in technology and the availability of data, implementing data analytics in sludge management has become increasingly feasible.
Implementing data analytics in sludge management offers numerous advantages. It enables wastewater treatment plants to optimize their operations, improve efficiency, and reduce costs. By analyzing data from various sources, such as sensors, monitoring systems, and historical records, plant operators can gain valuable insights into the sludge characteristics, treatment processes, and performance indicators.
Steps to Implement Data Analytics
The successful implementation of data analytics requires a well-defined roadmap. It starts with identifying goals, establishing data governance, ensuring data quality, selecting the appropriate tools, and building a capable team. Additionally, integrating analytics into existing workflows and promoting a data-driven culture are crucial elements of implementation.
Identifying goals is the first step in implementing data analytics in sludge management. This involves clearly defining the objectives and desired outcomes of the analytics initiative. Whether the goal is to optimize sludge treatment processes, improve energy efficiency, or enhance environmental sustainability, having a clear vision is essential.
Establishing data governance is another critical aspect of implementation. This involves defining data ownership, ensuring data privacy and security, and establishing data management protocols. By having a robust data governance framework in place, wastewater treatment plants can ensure the integrity and reliability of the data used for analytics.
Data quality is paramount in data analytics. To ensure accurate and reliable results, it is crucial to have high-quality data. This requires implementing data validation and verification processes, conducting regular data audits, and addressing any data inconsistencies or errors.
Selecting the appropriate tools is also essential in implementing data analytics in sludge management. There are various analytics tools available in the market, ranging from basic statistical software to advanced machine learning algorithms. The choice of tools depends on the specific requirements and capabilities of the wastewater treatment plant.
Building a capable team is crucial for the successful implementation of data analytics. This involves hiring or training personnel with the necessary skills and expertise in data analytics, statistics, and wastewater treatment. A multidisciplinary team comprising engineers, data scientists, and domain experts can bring diverse perspectives and ensure comprehensive analysis.
Integrating analytics into existing workflows is a key step in implementation. This involves identifying the points in the sludge management process where analytics can add value and integrating the analytics outputs into decision-making processes. By seamlessly integrating analytics into existing workflows, wastewater treatment plants can leverage the power of data to drive informed decisions.
Promoting a data-driven culture is essential for the long-term success of data analytics in sludge management. This involves creating awareness about the benefits of data-driven decision-making, providing training and support to employees, and fostering a culture of continuous learning and improvement.
Challenges in Implementing Data Analytics and How to Overcome Them
Implementing data analytics is not without its challenges. Some common hurdles include data compatibility issues, lack of skills and expertise, and resistance to change. Addressing these challenges requires investments in training and development, fostering a data-driven mindset, and selecting tools that integrate seamlessly with existing systems.
Data compatibility issues can arise when dealing with data from different sources or systems. In sludge management, data may come from various sensors, laboratory tests, and historical records. Ensuring data compatibility requires establishing data standards, implementing data integration techniques, and using data transformation tools.
Lack of skills and expertise in data analytics can be a significant barrier to implementation. Training and development programs can help bridge this gap by providing employees with the necessary knowledge and skills. Collaborating with external experts or partnering with universities can also bring in additional expertise and support.
Resistance to change is a common challenge when implementing data analytics in any industry. Overcoming resistance requires effective change management strategies, clear communication about the benefits of analytics, and involving stakeholders in the decision-making process. Demonstrating the value of data analytics through pilot projects or success stories can also help alleviate resistance.
In conclusion, implementing data analytics in sludge management is a complex but worthwhile endeavor. By following a well-defined roadmap, addressing challenges, and fostering a data-driven culture, wastewater treatment plants can unlock the full potential of data analytics and make informed decisions to optimize their sludge management processes.
Future Trends in Data Analytics for Sludge Management
As technology continues to advance, the field of data analytics in sludge management is poised for exciting developments. These trends hold promises that will revolutionize the industry and drive further improvements.
Predictive Analytics in Sludge Management
One of the most significant trends in data analytics is the adoption of predictive analytics in sludge management. By utilizing historical data, machine learning algorithms can forecast sludge production, track asset degradation, and optimize maintenance schedules. This predictive capability enables specialists to proactively manage their assets and prevent costly failures.
The Impact of Artificial Intelligence and Machine Learning on Data Analytics
Artificial intelligence (AI) and machine learning (ML) are revolutionizing the field of data analytics. AI-powered systems can automate data collection, analyze vast quantities of data, and provide actionable insights. ML algorithms can uncover hidden patterns, detect anomalies, and optimize processes. The integration of AI and ML into data analytics holds immense potential for driving efficiency and innovation in sludge management.
Conclusion: The Future of Data Analytics in Sludge Management
In conclusion, data analytics is becoming an essential tool for asset management specialists in the sludge management industry. By harnessing the power of data and leveraging advanced analytics techniques, specialists can optimize operations, enhance asset performance, and drive long-term sustainability. Embracing data analytics is not merely an option but a necessity to remain competitive and future-proof in the sludge management industry.
Key Takeaways
- Data analytics plays a crucial role in asset management in the sludge management industry.
- Implementing data analytics brings numerous benefits, including real-time monitoring and evidence-based decision-making.
- Fundamental concepts of data analytics include data collection, processing, and analysis.
- A wide range of data analytics tools is available, and selecting the right tool is essential.
- Implementing data analytics requires a strategic approach and overcoming challenges such as data compatibility and resistance to change.
- Predictive analytics and the integration of AI and ML are the future trends in data analytics for sludge management.
Final Thoughts on the Role of Data Analytics in Sludge Management
The future of sludge management lies in harnessing the power of data analytics. By adopting data-driven practices, asset management specialists can unlock hidden insights, optimize processes, and pave the way for a sustainable and efficient industry. Embracing data analytics is the pathway to transforming sludge management into a data-rich, insight-driven, and future-proof discipline.