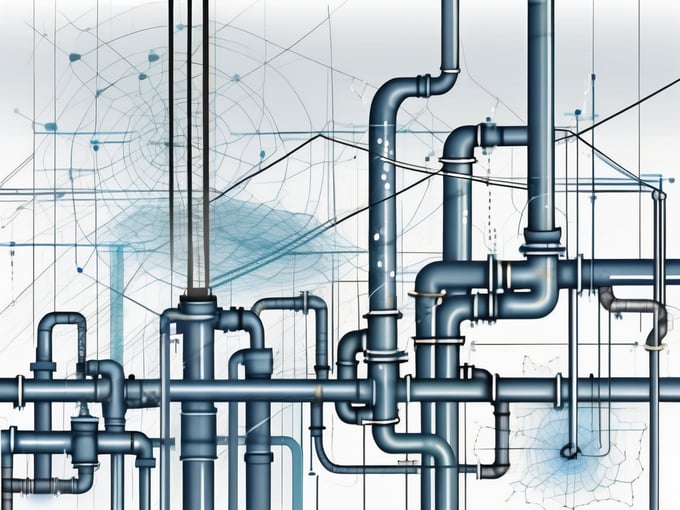
Important Trends in Data Analytics for Asset Management Specialists in the Stormwater Industry
Important Trends in Data Analytics for Asset Management Specialists in the Stormwater Industry
Data analytics has become an integral part of asset management in various industries, including the stormwater sector. As a stormwater asset management specialist, it is crucial to understand the role of data analytics and how it can improve the efficiency and effectiveness of your operations. In this comprehensive guide, we will explore the importance of data analytics in the stormwater industry, key concepts in data analytics for asset management, the intersection of data analytics and stormwater asset management, tools and techniques in data analytics, challenges and solutions in implementing data analytics, and future trends in this rapidly evolving field.
Understanding the Role of Data Analytics in Asset Management
Data analytics plays a vital role in asset management by providing valuable insights and facilitating data-driven decision-making. In the stormwater industry, data analytics enables asset management specialists to monitor, assess, and optimize the performance of stormwater infrastructure such as pipes, culverts, and detention ponds.
Stormwater infrastructure is critical for controlling and managing urban runoff, reducing flood risk, and protecting water quality. However, these assets are subject to aging, deterioration, and failure over time. Data analytics allows asset management specialists to identify patterns and trends in asset performance, prioritize maintenance and repair activities, and allocate resources effectively. By leveraging data analytics, stormwater asset management specialists can enhance the efficiency and reliability of their infrastructure, resulting in cost savings and improved service delivery.
One of the key benefits of data analytics in asset management is the ability to predict and prevent failures. By analyzing historical data and identifying patterns, asset management specialists can anticipate potential issues and take proactive measures to prevent failures. For example, if data analytics reveals a recurring pattern of pipe blockages in a particular area, preventive maintenance can be scheduled to clear the blockages and avoid potential flooding incidents.
Moreover, data analytics can also help optimize asset performance by identifying opportunities for improvement. By analyzing data on asset usage, maintenance activities, and performance indicators, asset management specialists can identify inefficiencies and implement strategies to enhance asset performance. For instance, if data analytics shows that a detention pond is frequently reaching its capacity during heavy rainfall events, asset management specialists can consider expanding the pond's capacity or implementing additional measures to improve its efficiency.
The Importance of Data Analytics in the Stormwater Industry
Stormwater infrastructure is critical for controlling and managing urban runoff, reducing flood risk, and protecting water quality. However, these assets are subject to aging, deterioration, and failure over time. Data analytics allows asset management specialists to identify patterns and trends in asset performance, prioritize maintenance and repair activities, and allocate resources effectively. By leveraging data analytics, stormwater asset management specialists can enhance the efficiency and reliability of their infrastructure, resulting in cost savings and improved service delivery.
One of the key challenges in stormwater asset management is the vast amount of data generated by various sources. Data analytics provides a solution to this challenge by enabling asset management specialists to process and analyze large datasets efficiently. By using advanced analytics techniques, such as machine learning algorithms, asset management specialists can extract valuable insights from the data and make informed decisions.
Furthermore, data analytics can also help in assessing the impact of climate change on stormwater infrastructure. By analyzing historical weather data and combining it with asset performance data, asset management specialists can identify potential vulnerabilities and develop strategies to mitigate the risks associated with climate change. For example, if data analytics reveals a significant increase in extreme rainfall events, asset management specialists can prioritize the maintenance and reinforcement of critical stormwater infrastructure to withstand such events.
Key Concepts in Data Analytics for Asset Management
To fully utilize data analytics in asset management, it is essential to understand key concepts and methodologies. For instance, asset performance indicators such as condition rating, failure frequency, and remaining useful life provide valuable insights into the health and performance of assets. These indicators can be derived from various data sources, including inspection reports, maintenance records, and sensor data. Additionally, statistical analysis techniques such as regression analysis and time series analysis can help identify underlying patterns and forecast future asset performance.
Another important concept in data analytics for asset management is data integration. Asset management specialists often deal with data from multiple sources, such as GIS data, sensor data, and maintenance records. Integrating these diverse datasets allows asset management specialists to gain a holistic view of asset performance and make more informed decisions. Data integration can be achieved through various techniques, including data cleansing, data transformation, and data fusion.
Furthermore, data visualization is a crucial aspect of data analytics in asset management. By presenting data in a visual format, such as charts, graphs, and maps, asset management specialists can easily interpret and communicate complex information. Data visualization helps in identifying trends, outliers, and patterns that may not be apparent in raw data. It also enables stakeholders to understand the implications of data analytics findings and make data-driven decisions.
The Intersection of Data Analytics and Stormwater Asset Management
Data analytics offers numerous opportunities for improving stormwater asset management practices. Two key areas where data analytics can make a significant impact are predictive analytics and efficient asset utilization.
The Role of Predictive Analytics in Stormwater Management
Predictive analytics leverages historical data, statistical models, and machine learning algorithms to forecast asset performance and identify potential failures before they occur. By applying predictive analytics to stormwater asset management, specialists can proactively prioritize maintenance and repair efforts, prevent costly reactive interventions, and extend the lifespan of critical infrastructure. Furthermore, predictive analytics enables more accurate budget forecasting and optimization, ensuring that limited resources are allocated where they are most needed.
For example, by analyzing historical data on rainfall patterns, water flow rates, and asset conditions, predictive analytics can identify areas at higher risk of flooding or infrastructure failure. This information allows stormwater management specialists to implement targeted maintenance and repair strategies, such as reinforcing vulnerable assets or clearing blocked drainage systems, before a major storm event occurs. By taking proactive measures based on predictive analytics, cities and municipalities can save significant costs associated with emergency repairs and minimize the disruption caused by flooding incidents.
Moreover, predictive analytics can also assist in predicting the long-term performance of stormwater assets. By analyzing data on asset deterioration rates, usage patterns, and environmental factors, specialists can forecast the remaining lifespan of infrastructure components. This information helps in developing long-term asset management plans and making informed decisions regarding asset replacement or rehabilitation. By extending the lifespan of critical stormwater infrastructure through predictive analytics, cities can achieve cost savings and ensure the reliability of their stormwater management systems.
Leveraging Data Analytics for Efficient Asset Utilization
Data analytics can also help stormwater asset management specialists optimize the utilization of their infrastructure. By analyzing data on asset usage, flow rates, and population growth, specialists can identify areas where capacity upgrades or new infrastructure investments are necessary. This proactive approach ensures that stormwater systems can cope with increasing demands, enhancing resilience and mitigating the risk of flooding. Additionally, data analytics enables optimization of asset maintenance schedules, minimizing disruptions and maximizing service delivery.
For instance, by analyzing data on population growth and urban development trends, stormwater management specialists can identify areas experiencing rapid expansion and increased stormwater runoff. By understanding these patterns, they can plan and implement infrastructure upgrades, such as constructing larger retention ponds or expanding drainage networks, to accommodate the growing demand. By leveraging data analytics to optimize asset utilization, cities can avoid overburdening existing infrastructure and ensure effective stormwater management in the face of urban growth.
Furthermore, data analytics can help in optimizing asset maintenance schedules. By analyzing historical data on asset performance, maintenance records, and repair costs, specialists can identify patterns and develop predictive maintenance models. These models can determine the optimal timing for asset inspections, cleaning, and repairs, minimizing the risk of failures and maximizing the lifespan of stormwater infrastructure. By implementing data-driven maintenance strategies, cities can reduce costs associated with reactive repairs and improve the overall performance and reliability of their stormwater systems.
Tools and Techniques in Data Analytics for Asset Management
Implementing data analytics in asset management requires specialized tools and techniques tailored to the unique challenges of the stormwater industry. In this article, we will explore some of the key tools and techniques used in data analytics for stormwater asset management.
Introduction to Data Analytics Tools for Asset Management
Various software applications enable stormwater asset management specialists to collect, store, analyze, and visualize data. One of the most commonly used tools is Geographic Information Systems (GIS) software. GIS software allows for spatial analysis and mapping of assets, facilitating informed decision-making. With GIS, asset managers can easily identify the location of assets, assess their condition, and plan maintenance activities accordingly.
In addition to GIS, data management platforms play a crucial role in effective asset management. These platforms enable efficient data integration, ensuring that multiple data sources can be effectively utilized. By consolidating data from various sources, asset managers can gain a comprehensive view of their assets and make data-driven decisions. Data management platforms also provide data cleansing and validation capabilities, ensuring the accuracy and reliability of the data used for analysis.
Furthermore, advanced analytics tools such as machine learning algorithms and artificial intelligence-driven models are increasingly being used in stormwater asset management. These tools enable the extraction of valuable insights from complex datasets, helping asset managers identify patterns, trends, and anomalies that may not be easily detectable through traditional analysis methods. By leveraging machine learning and AI, asset managers can predict asset failures, optimize maintenance schedules, and improve overall asset performance.
Advanced Techniques for Data Analysis in Stormwater Management
In addition to data analytics tools, advanced analytical techniques can further enhance stormwater asset management. For example, anomaly detection algorithms can detect abnormal patterns in data, alerting specialists to potential asset failures or performance deviations. By continuously monitoring data and comparing it to historical patterns, these algorithms can identify anomalies and trigger proactive maintenance actions, reducing the risk of asset failures and minimizing downtime.
Optimization algorithms are another powerful technique used in stormwater asset management. These algorithms assist in determining the most cost-effective maintenance strategies, considering factors such as asset criticality, accessibility, and budget constraints. By analyzing various maintenance scenarios and their associated costs, asset managers can make informed decisions on how to allocate their resources effectively. Optimization algorithms can also help in prioritizing maintenance activities based on the criticality of assets, ensuring that limited resources are utilized where they are most needed.
Bayesian networks offer a probabilistic approach to asset risk assessment in stormwater management. These networks model the relationships between different variables and their probabilities, allowing asset managers to make informed decisions based on uncertain data. By considering various factors such as asset condition, environmental conditions, and maintenance history, Bayesian networks can provide a holistic view of asset risk and help prioritize maintenance and rehabilitation efforts.
In conclusion, data analytics tools and advanced techniques play a crucial role in stormwater asset management. By leveraging these tools and techniques, asset managers can make data-driven decisions, optimize maintenance strategies, and improve the overall performance and resilience of stormwater assets.
Challenges and Solutions in Implementing Data Analytics
While the benefits of data analytics in stormwater asset management are significant, there are several challenges to overcome during implementation.
Data analytics has revolutionized the way organizations manage and utilize data. It enables them to extract valuable insights, make data-driven decisions, and optimize their operations. However, implementing data analytics comes with its own set of challenges that require careful consideration and planning.
Common Challenges in Data Analytics Implementation
Obtaining high-quality data is crucial for accurate and meaningful analysis. However, organizations often face challenges in collecting data that is complete, consistent, and reliable. Data may be scattered across various systems and databases, making it difficult to consolidate and integrate for analysis.
Ensuring data integrity is another challenge organizations face during data analytics implementation. Data integrity refers to the accuracy, consistency, and reliability of data. Inaccurate or inconsistent data can lead to flawed analysis and incorrect conclusions.
Data privacy and security concerns are also common challenges in data analytics implementation. Organizations need to ensure that sensitive data is protected and comply with relevant data protection regulations. This involves implementing robust security measures, such as encryption and access controls, to safeguard data from unauthorized access or breaches.
Integrating data from multiple systems and sources can be complex and time-consuming. Organizations often have data stored in different formats and databases, making it challenging to bring them together for analysis. Data integration requires careful planning, mapping, and transformation to ensure that data from various sources can be effectively combined.
Data analytics also requires specialized skills and knowledge. Organizations may lack the necessary expertise in-house, necessitating training or collaboration with data analytics experts. Without the right skills, organizations may struggle to effectively analyze and interpret data, limiting the potential benefits of data analytics.
Effective Solutions for Data Analytics Challenges
To address these challenges, organizations need to establish robust data governance practices. This includes defining data quality standards and implementing validation processes to ensure that data is accurate and reliable. Data access controls should also be put in place to restrict access to sensitive data and protect privacy.
Implementing secure and scalable data storage and processing infrastructure is essential for successful data analytics implementation. Organizations should invest in robust technologies and platforms that can handle large volumes of data securely. This includes implementing backup and disaster recovery measures to ensure data availability and continuity.
Collaboration with data analytics experts or partnering with specialized service providers can bridge knowledge gaps and accelerate implementation. These experts can provide guidance and support in data integration, analysis, and interpretation. They can also help organizations develop data analytics strategies and roadmaps to maximize the value of their data.
Continuous training and upskilling of staff are crucial to ensure that the organization remains adept at leveraging data analytics technologies. Organizations should invest in training programs and workshops to enhance the data analytics skills of their employees. This will enable them to effectively use data analytics tools and techniques to extract insights and drive data-driven decision-making.
In conclusion, implementing data analytics in stormwater asset management offers numerous benefits, but it also comes with its own set of challenges. By addressing these challenges through robust data governance, secure infrastructure, collaboration with experts, and continuous training, organizations can successfully implement data analytics and unlock the full potential of their data.
Future Trends in Data Analytics for Stormwater Asset Management
Data analytics in stormwater asset management is an exciting field that continues to evolve. Several promising trends offer future opportunities for asset management specialists.
Emerging Trends in Data Analytics
One emerging trend is the use of real-time data from sensors and IoT devices, enabling continuous monitoring and immediate response to changing conditions. This real-time data opens up possibilities for dynamic decision-making, predictive maintenance, and enhanced asset performance optimization. Furthermore, the integration of data analytics with geospatial technologies and remote sensing offers new insights into asset conditions and helps identify spatial patterns that impact asset performance.
The Future of Asset Management in the Stormwater Industry
Looking ahead, asset management in the stormwater industry will increasingly rely on data-driven approaches. Advanced analytics techniques such as artificial intelligence, machine learning, and deep learning will play a vital role in extracting insights from vast amounts of data. The ability to combine data from different sources, such as weather data or social media feeds, will provide a holistic view of asset performance and risks. Additionally, predictive analytics capabilities will continue to improve, allowing for more accurate forecasting of asset behavior and performance.
In conclusion, data analytics is revolutionizing asset management in the stormwater industry. By understanding the role of data analytics, key concepts, and techniques, asset management specialists can improve their decision-making and optimize the performance of their infrastructure. Despite the challenges, implementing data analytics can lead to cost savings, improved service delivery, and enhanced resilience. As the field continues to evolve, staying abreast of emerging trends and leveraging advanced analytics capabilities will be crucial for asset management specialists in the stormwater industry.