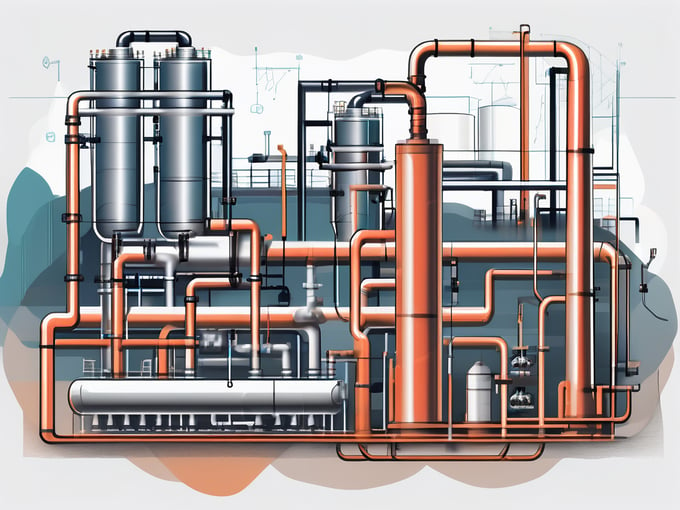
Important Trends in Data Analytics for Consulting Engineers in the Sludge Management Industry
Data analytics has become an essential tool for consulting engineers in the sludge management industry. With the vast amounts of data available in this field, it is crucial for engineers to harness its potential to drive efficiency and make informed decisions. In this ultimate guide, we will explore the role of data analytics in sludge management, key techniques to apply, implementation strategies, tools and software options, measuring the impact, and future trends. By the end of this guide, consulting engineers will have a comprehensive understanding of how to leverage data analytics to revolutionize their sludge management operations.
Understanding the Role of Data Analytics in Sludge Management
Defining Data Analytics in the Context of Sludge Management
Data analytics refers to the process of extracting insights and patterns from data to make informed decisions. In the context of sludge management, data analytics involves analyzing various data sources, including sensor data, historic records, and operational data, to gain valuable insights into the sludge composition, treatment processes, and overall system performance.
When it comes to sludge management, data analytics plays a crucial role in understanding the complex nature of sludge and its treatment. By applying advanced statistical and mathematical techniques, data analytics allows us to uncover hidden patterns and correlations within the data, providing us with a deeper understanding of the sludge management process.
One of the key aspects of data analytics in sludge management is the analysis of sensor data. Sensors placed at different stages of the sludge treatment process collect valuable information about the sludge's physical and chemical properties. By analyzing this sensor data, we can monitor the sludge's characteristics in real-time, identify any deviations from the desired parameters, and take corrective actions promptly.
Moreover, data analytics also involves analyzing historic records and operational data. Historic records provide us with a wealth of information about the sludge's composition, treatment methods used in the past, and their effectiveness. By studying this historical data, we can identify trends and patterns that can help us optimize the current sludge management practices.
The Importance of Data Analytics for Consulting Engineers
For consulting engineers in the sludge management industry, data analytics offers numerous benefits. It enables engineers to optimize processes, predict system failures, identify areas for improvement, and make data-driven recommendations to clients. By leveraging data analytics, consulting engineers can unlock new opportunities for efficiency, cost-saving, and sustainable sludge management practices.
Optimizing processes is a key objective for consulting engineers in the sludge management industry. By analyzing data from various sources, engineers can identify bottlenecks, inefficiencies, and areas where improvements can be made. For example, data analytics can help identify the optimal dosage of chemicals required for sludge treatment, reducing costs and minimizing environmental impact.
In addition to process optimization, data analytics also plays a crucial role in predicting system failures. By analyzing historical data and monitoring real-time sensor data, consulting engineers can identify early warning signs of potential system failures. This proactive approach allows engineers to take preventive measures, avoiding costly downtime and ensuring uninterrupted sludge treatment operations.
Furthermore, data analytics empowers consulting engineers to make data-driven recommendations to their clients. By analyzing data and extracting meaningful insights, engineers can provide evidence-based recommendations for improving sludge management practices. These recommendations may include adopting new treatment technologies, implementing process modifications, or optimizing resource allocation.
Overall, data analytics is a powerful tool for consulting engineers in the sludge management industry. It enables engineers to gain a deeper understanding of the sludge treatment process, optimize operations, predict system failures, and make informed recommendations to clients. By harnessing the power of data analytics, consulting engineers can drive innovation, efficiency, and sustainability in sludge management practices.
Key Data Analytics Techniques for Sludge Management
Predictive Analytics for Future Sludge Management Strategies
Predictive analytics uses historical data and statistical models to forecast future trends and outcomes. In sludge management, consulting engineers can utilize predictive analytics to anticipate sludge generation rates, estimate treatment plant capacity requirements, and develop proactive maintenance schedules. By accurately predicting sludge management needs, engineers can optimize resource allocation and prevent costly disruptions.
One example of how predictive analytics can be applied in sludge management is in predicting the growth rate of sludge in treatment plants. By analyzing historical data on sludge generation, engineers can develop models that take into account various factors such as population growth, industrial activities, and weather patterns to forecast future sludge generation rates. This information is crucial for planning the expansion of treatment plant capacity and ensuring that the infrastructure can handle the projected increase in sludge volume.
Another application of predictive analytics in sludge management is in developing proactive maintenance schedules. By analyzing historical data on equipment failures and maintenance activities, engineers can identify patterns and correlations that can help predict when equipment is likely to fail. This allows for timely maintenance interventions, reducing the risk of equipment breakdowns and minimizing downtime in the treatment process.
Descriptive Analytics for Current Sludge Management Assessment
Descriptive analytics focuses on summarizing and interpreting past data to gain insights into current performance. For consulting engineers in sludge management, descriptive analytics helps in assessing the efficiency of treatment processes, identifying bottlenecks, and measuring compliance with regulatory standards. By understanding the current state of sludge management operations, engineers can develop targeted improvement strategies.
One way descriptive analytics can be used in sludge management is in assessing the efficiency of treatment processes. By analyzing data on key performance indicators such as sludge removal efficiency, treatment time, and energy consumption, engineers can identify areas where improvements can be made. For example, if the data shows that a particular treatment process is less efficient compared to industry standards, engineers can investigate the root causes and implement corrective measures to optimize performance.
Descriptive analytics also plays a crucial role in measuring compliance with regulatory standards in sludge management. By analyzing data on parameters such as sludge composition, heavy metal concentrations, and pathogen levels, engineers can ensure that the treatment process meets the required standards before the sludge is disposed of or reused. This helps prevent environmental contamination and ensures that the sludge is safely managed according to regulations.
Implementing Data Analytics in Sludge Management Operations
Data analytics is becoming increasingly important in the field of sludge management operations. By harnessing the power of data, consulting engineers can optimize their workflows, improve efficiency, and make informed decisions. In this article, we will explore the steps to incorporate data analytics in sludge management operations and discuss the challenges that may arise during implementation.
Steps to Incorporate Data Analytics in Your Workflow
To effectively implement data analytics in sludge management operations, consulting engineers should follow a structured approach:
- Identify the data sources and types to be collected: The first step is to identify the relevant data sources and determine the types of data that need to be collected. This may include data from sensors, laboratory tests, operational logs, and maintenance records.
- Design a data collection and storage system: Once the data sources have been identified, a robust data collection and storage system should be designed. This system should ensure the secure and efficient storage of data, allowing for easy retrieval and analysis.
- Pre-process and cleanse data for quality assurance: Raw data often contains errors, outliers, and missing values. Before analysis, it is crucial to pre-process and cleanse the data to ensure its quality and reliability. This may involve data cleaning, normalization, and outlier detection techniques.
- Explore and identify relevant variables for analysis: After data pre-processing, it is important to explore the data and identify the variables that are relevant to the analysis. This may involve statistical techniques, data visualization, and domain knowledge.
- Apply appropriate analytics techniques: Once the relevant variables have been identified, consulting engineers can apply appropriate analytics techniques to gain insights from the data. This may include descriptive statistics, predictive modeling, and machine learning algorithms.
- Interpret and communicate the results: The insights obtained from data analytics need to be interpreted and communicated effectively. Consulting engineers should be able to explain the implications of the results to stakeholders and decision-makers, using visualizations, reports, and presentations.
- Implement recommended changes and monitor the impact: Finally, the recommended changes based on the data analytics results should be implemented in the sludge management operations. It is important to monitor the impact of these changes and continuously evaluate the effectiveness of the data-driven decisions.
Overcoming Challenges in Data Analytics Implementation
Implementing data analytics in sludge management comes with its challenges. These challenges may include:
- Data quality issues: The quality of data can significantly impact the accuracy and reliability of the analytics results. Incomplete, inconsistent, or erroneous data can lead to misleading insights. It is important to address data quality issues through data cleansing, validation, and verification processes.
- Limited resources for data collection and analysis: Collecting and analyzing data requires resources, including personnel, technology, and infrastructure. Limited resources can pose a challenge in implementing data analytics. Consulting engineers can overcome this challenge by prioritizing data collection efforts, leveraging existing resources, and seeking partnerships or collaborations.
- Resistance to change: Introducing data analytics into established workflows may face resistance from stakeholders who are accustomed to traditional methods. It is important to address this resistance by demonstrating the value and benefits of data analytics, providing training and support, and involving stakeholders in the decision-making process.
To address these challenges, consulting engineers can invest in data management systems that facilitate data collection, storage, and analysis. Adopting data governance frameworks can ensure data quality, privacy, and security. Furthermore, fostering a culture of data-driven decision-making within organizations can encourage the adoption of data analytics practices and overcome resistance to change.
Tools and Software for Data Analytics in Sludge Management
Overview of Popular Data Analytics Tools
Consulting engineers have access to a range of data analytics tools that can greatly aid in the management of sludge. These tools provide the means to efficiently analyze and manipulate data, enabling engineers to make informed decisions and optimize sludge management processes. Some well-known tools in the field of data analytics include:
- Python and R: These programming languages are widely used for data manipulation and analysis. They offer a wide range of libraries and packages specifically designed for statistical analysis, making them powerful tools for sludge management.
- Excel: As a spreadsheet software, Excel is commonly used for basic data analysis and visualization. Its user-friendly interface and familiar layout make it accessible to engineers with varying levels of expertise.
- Tableau and Power BI: These data visualization tools are highly effective in creating interactive dashboards and visual representations of data. They allow engineers to present complex information in a visually appealing and easily understandable manner.
- Statistical Packages: Software such as SPSS and SAS are specifically designed for advanced statistical analysis. These packages provide a wide range of statistical functions and tools that can be utilized in sludge management projects.
Choosing the Right Software for Your Needs
When selecting data analytics software for sludge management, consulting engineers should carefully consider various factors to ensure the chosen tool aligns with their specific requirements. Some key factors to consider include:
- Expertise Level: Engineers should assess their own proficiency in using different tools and choose software that matches their skillset. This ensures that they can effectively utilize the features and functionalities of the selected tool.
- Specific Analysis Requirements: Different sludge management projects may have unique analysis requirements. Engineers should evaluate the capabilities of each software in meeting these specific needs, such as data cleaning, modeling, and predictive analytics.
- Integration Capabilities: It is crucial to consider the compatibility and integration capabilities of the software with existing systems and databases. Seamless integration allows for efficient data transfer and avoids potential data inconsistencies.
- Scalability: As sludge management projects often involve large volumes of data, engineers should choose software that can handle the scale of their data. Scalability ensures that the software can effectively process and analyze data without compromising performance.
Evaluating the suitability of different tools for sludge management projects can be done through various methods. Engineers can explore trial versions or demos provided by software vendors to get a hands-on experience of the tools. Additionally, consulting with software vendors and seeking their expertise can provide valuable insights into the best software options for specific sludge management needs.
Measuring the Impact of Data Analytics on Sludge Management
Sludge management is a critical aspect of wastewater treatment, and the use of data analytics has revolutionized the way consulting engineers approach this task. By analyzing and interpreting large volumes of data, engineers can make informed decisions that optimize sludge management practices and improve overall efficiency.
Key Performance Indicators for Data Analytics Success
To measure the impact of data analytics in sludge management, consulting engineers can track a range of key performance indicators (KPIs). These KPIs provide valuable insights into the effectiveness of data analytics initiatives. Some of the key performance indicators include:
- Sludge treatment efficiency rate: This KPI measures the effectiveness of the treatment process in removing impurities from the sludge. By monitoring this indicator, engineers can identify areas for improvement and implement necessary changes.
- Reduction in waste disposal costs: Data analytics can help identify opportunities to reduce waste disposal costs by optimizing the treatment process and minimizing the amount of sludge generated.
- Mean time between failures of treatment systems: By analyzing historical data, engineers can predict the frequency of system failures and plan maintenance activities accordingly. This KPI helps in minimizing downtime and ensuring uninterrupted sludge management operations.
- Energy consumption reduction: Data analytics can identify energy-intensive processes and suggest ways to optimize energy usage, leading to cost savings and reduced environmental impact.
- Compliance with environmental regulations: By monitoring and analyzing data related to environmental parameters, engineers can ensure that sludge management practices comply with regulatory standards, minimizing the risk of penalties and environmental harm.
By regularly monitoring these KPIs, consulting engineers can evaluate the effectiveness of their data analytics initiatives and make necessary adjustments to optimize sludge management practices. The insights gained from data analytics enable engineers to make data-driven decisions, resulting in improved overall performance.
Long-Term Benefits of Data-Driven Sludge Management
The adoption of data analytics in sludge management can lead to significant long-term benefits for both the industry and the environment. Some of these benefits include:
- Improved operational efficiency: Data analytics enables engineers to identify bottlenecks and inefficiencies in the sludge management process. By addressing these issues, operational efficiency can be improved, resulting in cost savings and enhanced performance.
- Cost savings through optimized resource allocation: By analyzing data on resource utilization, engineers can optimize the allocation of manpower, equipment, and materials. This leads to cost savings and improved resource management.
- Enhanced environmental sustainability: Data analytics helps in identifying opportunities for reducing the environmental impact of sludge management. By optimizing treatment processes and minimizing waste generation, the industry can contribute to a more sustainable future.
- Enhanced regulatory compliance: Compliance with environmental regulations is a crucial aspect of sludge management. Data analytics enables engineers to monitor and ensure compliance, reducing the risk of legal and environmental consequences.
- Proactive maintenance and reduced downtime: By analyzing data on equipment performance and maintenance history, engineers can implement proactive maintenance strategies. This helps in reducing downtime, improving system reliability, and minimizing disruptions in sludge management operations.
By leveraging the power of data, consulting engineers can transform the sludge management industry into a more efficient, sustainable, and cost-effective space for all stakeholders. The insights gained from data analytics enable informed decision-making, leading to continuous improvement and better outcomes in sludge management.
Future Trends in Data Analytics for Sludge Management
Emerging Technologies in Data Analytics
The field of data analytics is constantly evolving, and consulting engineers should stay updated on emerging technologies. Some notable trends include:
- Internet of Things (IoT): Integration of sensors and real-time data collection devices for enhanced monitoring and automation.
- Machine Learning: Algorithms that adapt and improve over time, enabling more accurate predictions and decision-making.
- Artificial Intelligence: Systems that can analyze extensive datasets to identify patterns and provide valuable insights.
By embracing these emerging technologies, consulting engineers can stay at the forefront of sludge management innovation and drive continuous improvement.
The Future of Sludge Management with Data Analytics
The future of sludge management lies in the integration of data analytics as a core part of the industry. Consulting engineers will increasingly rely on data-driven decision-making, leveraging advanced analytics techniques and emerging technologies to optimize processes, improve environmental sustainability, and meet the growing demands of an evolving landscape. As data analytics continues to evolve, it will revolutionize the sludge management industry, allowing consulting engineers to address challenges and unlock new opportunities for a cleaner, more efficient future.
In conclusion, data analytics is becoming increasingly vital for consulting engineers in the sludge management industry. It offers numerous benefits, including predictive and descriptive analytics techniques, implementation strategies, suitable tools and software options, methods to measure impact, and future trends. By harnessing the power of data analytics, consulting engineers can drive efficiency, optimize resources, and make informed decisions that positively impact sludge management operations. Embracing data analytics is essential for consulting engineers looking to stay ahead in the rapidly evolving sludge management industry.