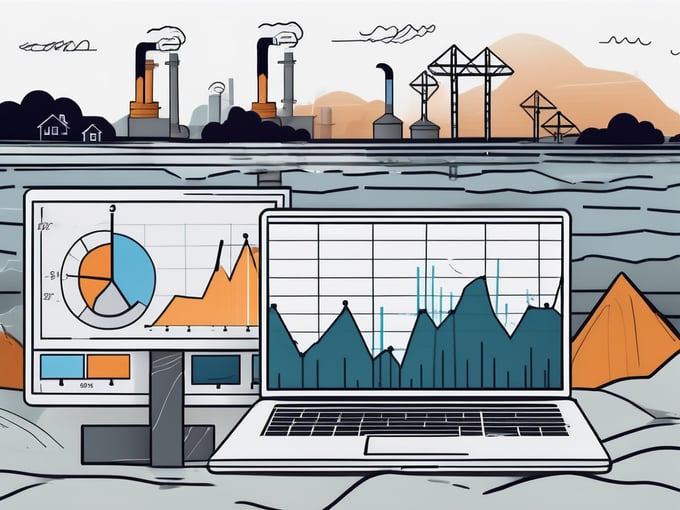
Important Trends in Data Analytics for Consulting Engineers in the Stormwater Industry
In the ever-evolving field of consulting engineering in the stormwater industry, data analytics has emerged as a powerful tool for managing and optimizing stormwater management systems. By harnessing the power of data, consulting engineers can gain valuable insights and make informed decisions that can lead to more efficient and effective stormwater management strategies. In this ultimate guide, we will explore the importance of data analytics in the stormwater industry, the key concepts, implementation steps, tools and technologies, measuring impact, and future trends in data analytics.
Understanding the Importance of Data Analytics in the Stormwater Industry
Stormwater management plays a crucial role in mitigating flooding, preserving water quality, and protecting the environment. However, the traditional approaches to stormwater management often fall short in addressing the complex challenges presented by urbanization and climate change. This is where data analytics steps in.
Data analytics involves the collection, analysis, and interpretation of large volumes of data to uncover patterns, trends, and relationships. In the context of stormwater management, data analytics can help consulting engineers gain a deeper understanding of how stormwater systems behave, identify areas of concern or potential risks, and develop data-driven strategies to address them.
By utilizing data analytics, consulting engineers can optimize stormwater management systems to ensure their effectiveness in handling the increasing demands of urbanization and climate change. With the ability to analyze vast amounts of data, engineers can identify patterns and trends that may not be apparent through traditional approaches. This enables them to make more informed decisions and develop innovative solutions to complex stormwater challenges.
One of the key benefits of data analytics in stormwater management is its ability to predict and prevent flooding events. By analyzing historical weather data, topography, and the behavior of stormwater systems, engineers can develop predictive models that anticipate potential flooding areas. This allows for proactive measures to be taken, such as implementing additional drainage infrastructure or modifying existing systems to handle increased water flow.
Data analytics also plays a crucial role in optimizing pollutant control measures in stormwater management. By analyzing water quality data and identifying pollutant sources, engineers can develop targeted strategies to reduce contamination. This may involve implementing green infrastructure solutions, such as rain gardens or permeable pavements, to filter pollutants before they enter stormwater systems. By optimizing pollutant control measures, data analytics helps to protect water quality and minimize the impact of stormwater runoff on the environment.
Furthermore, data analytics enables consulting engineers to ensure compliance with regulatory requirements. By analyzing data on stormwater quality and quantity, engineers can assess whether stormwater management systems meet the standards set by local, state, and federal regulations. This ensures that stormwater infrastructure is designed and maintained in accordance with the necessary guidelines, helping to protect public health and the environment.
In conclusion, data analytics is a powerful tool in the stormwater industry. It empowers consulting engineers to gain valuable insights, make informed decisions, and develop innovative solutions to the challenges posed by urbanization and climate change. By leveraging data analytics, engineers can optimize stormwater management systems, predict and prevent flooding events, optimize pollutant control measures, and ensure compliance with regulatory requirements. As the field of data analytics continues to advance, its role in stormwater management will only become more crucial in creating sustainable and resilient communities.
Key Concepts in Data Analytics for the Stormwater Industry
Before diving into the implementation of data analytics in your engineering practice, it is essential to familiarize yourself with the key concepts and terminology. Let's explore two fundamental concepts that form the foundation of data analytics in the stormwater industry.
Introduction to Data Analytics Terminology: To navigate the world of data analytics, it's important to understand key terms such as data mining, data visualization, descriptive analysis, inferential analysis, and machine learning. These concepts play a crucial role in uncovering patterns, generating insights, and making data-driven decisions in the stormwater industry.
Data mining involves extracting valuable information and patterns from large datasets. It helps identify hidden relationships and trends that can be used to improve stormwater management strategies. By applying data mining techniques, engineers can discover patterns in rainfall data, pollutant levels, and water flow rates, enabling them to make more informed decisions.
Data visualization is the process of presenting data in a visual format, such as charts, graphs, and maps. It allows engineers to communicate complex information effectively and identify patterns or anomalies at a glance. Through interactive visualizations, stakeholders can gain a better understanding of stormwater data, enabling them to collaborate and make informed decisions together.
Descriptive analysis involves summarizing and interpreting data to gain insights into past events or trends. By analyzing historical stormwater data, engineers can identify patterns, understand the impact of previous management strategies, and assess the effectiveness of stormwater infrastructure. This information is crucial for developing more efficient and sustainable stormwater management plans.
Inferential analysis, on the other hand, involves drawing conclusions or making predictions based on a sample of data. By applying statistical techniques to a subset of stormwater data, engineers can make inferences about the entire stormwater system. This allows them to estimate future rainfall patterns, predict potential flooding risks, and evaluate the impact of different management scenarios.
Machine learning is a branch of artificial intelligence that focuses on developing algorithms capable of learning from and making predictions or decisions based on data. In the stormwater industry, machine learning algorithms can be trained to recognize patterns in historical data and use that knowledge to predict future events or optimize stormwater management strategies. By leveraging machine learning, engineers can automate data analysis processes and enhance the accuracy of their predictions.
Understanding Predictive Analysis in Stormwater Management: Predictive analysis involves using historical data to forecast future events or behaviors. In the context of stormwater management, predictive analysis can be utilized to predict rainfall patterns, flooding risks, and water quality changes. By harnessing predictive analysis, consulting engineers can proactively plan and implement measures to mitigate potential risks.
By analyzing historical rainfall data, engineers can identify seasonal patterns and trends, allowing them to anticipate periods of heavy rainfall and potential flooding risks. This information is crucial for designing stormwater infrastructure that can handle increased water flow during extreme weather events, reducing the risk of flooding and property damage.
Predictive analysis can also be applied to water quality monitoring. By analyzing historical data on pollutant levels and water quality parameters, engineers can identify potential sources of contamination and predict changes in water quality. This enables them to implement targeted measures to improve water quality and protect ecosystems.
Furthermore, predictive analysis can help optimize stormwater management strategies by simulating different scenarios and predicting their outcomes. By inputting various parameters, such as land use, rainfall intensity, and stormwater infrastructure characteristics, engineers can evaluate the effectiveness of different management strategies and identify the most efficient and cost-effective solutions.
In conclusion, understanding key concepts and terminology in data analytics is crucial for implementing effective stormwater management strategies. By leveraging data mining, data visualization, descriptive and inferential analysis, and machine learning, engineers can uncover patterns, generate insights, and make data-driven decisions. Additionally, predictive analysis plays a vital role in anticipating future events and behaviors, enabling engineers to proactively plan and implement measures to mitigate risks in the stormwater industry.
Implementing Data Analytics in Your Engineering Practice
While the potential benefits of data analytics in the stormwater industry are vast, implementing data analytics into your engineering practice requires careful planning and execution. Let's explore the steps to successfully incorporate data analytics into your consulting engineering practice.
Data analytics has revolutionized various industries, including stormwater management. By leveraging the power of data, engineers can gain valuable insights and make informed decisions to improve the efficiency and effectiveness of their projects. However, the process of implementing data analytics is not as simple as it may seem. It involves several crucial steps that need to be followed diligently to ensure success.
- Step 1: Define Objectives and Identify Data Needs: Clearly define your objectives for leveraging data analytics in stormwater management. Identify the specific data requirements that align with your objectives, such as rainfall data, water level measurements, or pollutant concentrations.
Defining clear objectives is essential to ensure that your data analytics efforts are focused and targeted. By identifying the specific data needs, you can streamline the data collection process and avoid wasting resources on irrelevant data.
- Step 2: Collect and Organize Data: Gather the relevant data from various sources, including monitoring stations, sensors, and historical records. Ensure the data is accurate, complete, and properly structured for analysis.
Data collection is a critical step in the data analytics process. It involves gathering data from various sources, such as monitoring stations and sensors, to create a comprehensive dataset. The accuracy and completeness of the data are crucial for obtaining reliable insights.
- Step 3: Data Preprocessing and Cleaning: Prepare the data by removing duplicates, handling missing values, and standardizing formats. This step is crucial for ensuring the accuracy and reliability of the analysis.
Data preprocessing and cleaning are essential to ensure that the data is in a suitable format for analysis. This step involves removing duplicate entries, handling missing values, and standardizing data formats. By cleaning the data, engineers can eliminate any potential biases or errors that may affect the analysis.
- Step 4: Data Analysis and Modeling: Apply appropriate data analysis techniques, such as statistical methods or machine learning algorithms, to extract meaningful insights from the data. Develop models that can predict future events or optimize decision-making processes.
Data analysis and modeling are at the core of data analytics. By applying statistical methods or machine learning algorithms, engineers can uncover patterns, trends, and correlations in the data. These insights can then be used to make informed decisions and improve the efficiency of stormwater management practices.
- Step 5: Interpretation and Visualization: Interpret the results of the data analysis and present them in a clear and visually appealing manner. Visualization tools, such as charts, graphs, and maps, can help communicate complex information effectively.
Interpreting the results of data analysis is crucial for deriving actionable insights. By presenting the findings in a clear and visually appealing manner, engineers can effectively communicate complex information to stakeholders. Visualization tools, such as charts, graphs, and maps, can enhance understanding and facilitate decision-making processes.
Implementing data analytics in the stormwater industry is not without its challenges. Common challenges include data quality issues, lack of domain expertise, and limited resources. However, with proper planning, collaboration with domain experts, and investment in reliable data collection infrastructure, these challenges can be overcome.
Data quality is a significant concern in data analytics. Inaccurate or incomplete data can lead to misleading insights and flawed decision-making. To address this challenge, engineers should establish robust data quality assurance processes, including data validation and verification techniques. Collaborating with domain experts, such as hydrologists and environmental scientists, can also help ensure that the data analysis is grounded in sound scientific principles.
Lack of domain expertise is another common challenge faced during the implementation of data analytics. Data analytics requires a deep understanding of the industry and its specific challenges. To overcome this challenge, engineers can seek partnerships with experts in the field or invest in training programs to enhance their knowledge and skills in data analytics.
Limited resources, both in terms of budget and technology, can hinder the implementation of data analytics. However, with careful planning and prioritization, engineers can make the most of the available resources. Investing in reliable data collection infrastructure, such as advanced sensors and monitoring systems, can also help ensure the availability of high-quality data for analysis.
In conclusion, implementing data analytics in your engineering practice can bring numerous benefits to the stormwater industry. By following the steps outlined above and addressing the challenges along the way, engineers can harness the power of data to make informed decisions, optimize processes, and improve the overall efficiency of stormwater management practices.
Tools and Technologies in Data Analytics for Stormwater Industry
The field of data analytics is rapidly evolving, and new tools and technologies are constantly emerging to support the analysis and interpretation of data in the stormwater industry. Let's explore some key tools and technologies that consulting engineers can leverage to enhance their data analytics capabilities.
Overview of Data Analytics Tools: There are numerous data analytics tools available that cater specifically to the needs of the stormwater industry. These tools offer features such as data integration, analysis, visualization, and reporting. Popular tools include ArcGIS, MATLAB, R, Python, and Excel.
ArcGIS: ArcGIS is a powerful geographic information system (GIS) software that allows consulting engineers to analyze and visualize spatial data related to stormwater management. With ArcGIS, engineers can create interactive maps, perform spatial analysis, and generate reports to gain valuable insights into stormwater patterns and trends. This tool enables efficient decision-making by providing a comprehensive view of stormwater data.
MATLAB: MATLAB is a high-level programming language and environment that is widely used in data analytics. It offers a range of tools and functions for data manipulation, visualization, and statistical analysis. Consulting engineers can leverage MATLAB to analyze large datasets, develop complex models, and perform advanced statistical analysis to identify patterns and correlations in stormwater data.
R: R is a popular open-source programming language and software environment for statistical computing and graphics. It provides a wide range of statistical and graphical techniques for data analysis, making it an ideal tool for consulting engineers working with stormwater data. R allows engineers to perform data manipulation, visualization, and modeling, and it also offers a vast collection of packages for specialized data analysis tasks.
Python: Python is a versatile programming language that is widely used in data analytics. It offers a rich set of libraries and frameworks for data manipulation, analysis, and visualization. Consulting engineers can leverage Python to clean and preprocess stormwater data, perform statistical analysis, and create interactive visualizations to communicate their findings effectively.
Excel: Excel is a widely used spreadsheet program that offers basic data analysis capabilities. While it may not be as powerful as specialized data analytics tools, Excel is still a valuable tool for consulting engineers working with stormwater data. It allows engineers to organize and manipulate data, perform basic calculations and statistical analysis, and create charts and graphs to visualize the data.
Emerging Technologies in Data Analytics: With advancements in technology, consulting engineers can now leverage emerging technologies such as Internet of Things (IoT), remote sensing, and artificial intelligence (AI) to enhance their data analytics capabilities. These technologies enable real-time data collection, automate data analysis processes, and provide predictive insights for improved decision-making.
Internet of Things (IoT): IoT refers to the network of physical devices, vehicles, and other objects embedded with sensors, software, and connectivity, enabling them to collect and exchange data. In the stormwater industry, IoT devices can be deployed to monitor various parameters such as water levels, flow rates, and water quality in real-time. This data can then be analyzed to identify potential issues, optimize stormwater management strategies, and improve overall system efficiency.
Remote Sensing: Remote sensing involves the collection of data about the Earth's surface from a distance, typically using satellites or aircraft. In the context of stormwater management, remote sensing can be used to gather information about land cover, vegetation, and other relevant factors that influence stormwater runoff. This data can then be integrated with other datasets to gain a comprehensive understanding of stormwater patterns and make informed decisions.
Artificial Intelligence (AI): AI refers to the simulation of human intelligence in machines that are programmed to think and learn like humans. In the stormwater industry, AI can be used to analyze large volumes of data, identify patterns and anomalies, and make predictions about future stormwater events. AI algorithms can learn from historical data and continuously improve their performance, enabling consulting engineers to make data-driven decisions and optimize stormwater management strategies.
By leveraging these tools and technologies, consulting engineers in the stormwater industry can unlock the full potential of their data and gain valuable insights that can drive informed decision-making and improve overall stormwater management practices.
Measuring the Impact of Data Analytics on Stormwater Management
Implementing data analytics in the stormwater industry has the potential to significantly impact the efficiency and effectiveness of stormwater management practices. Let's explore how the impact of data analytics can be measured and evaluated.
Key Performance Indicators for Data Analytics: Key performance indicators (KPIs) can be used to assess the effectiveness and efficiency of data analytics initiatives. KPIs may include metrics such as reduction in flooding incidents, improved water quality, cost savings, and increased regulatory compliance.
Long-term Benefits of Data Analytics in Stormwater Management: Beyond the immediate impact, data analytics in the stormwater industry offers long-term benefits such as improved resilience to climate change, informed infrastructure planning, and better resource allocation. These long-term benefits contribute to the overall sustainability of stormwater management systems.
Future Trends in Data Analytics for the Stormwater Industry
As technology continues to advance and our understanding of data analytics deepens, it's important for consulting engineers in the stormwater industry to stay ahead of the curve. Let's explore some predicted developments and future trends in data analytics for stormwater management.
Predicted Developments in Data Analytics: The future of data analytics in the stormwater industry is expected to include advancements in machine learning algorithms, improved data collection technologies, and increased integration of artificial intelligence. These developments will further enhance the accuracy, efficiency, and scalability of data analytics practices.
Preparing for the Future of Data Analytics in Stormwater Management: To prepare for the future of data analytics in the stormwater industry, consulting engineers should focus on continuous learning and professional development. Familiarize yourself with emerging technologies, stay updated on industry trends, and actively seek opportunities to incorporate cutting-edge data analytics techniques into your practice.
By harnessing the power of data analytics, consulting engineers in the stormwater industry can revolutionize stormwater management practices and pave the way for a more sustainable future. Whether you are just starting your data analytics journey or looking to further enhance your existing capabilities, this ultimate guide serves as a comprehensive resource to help you navigate the exciting world of data analytics in the stormwater industry.