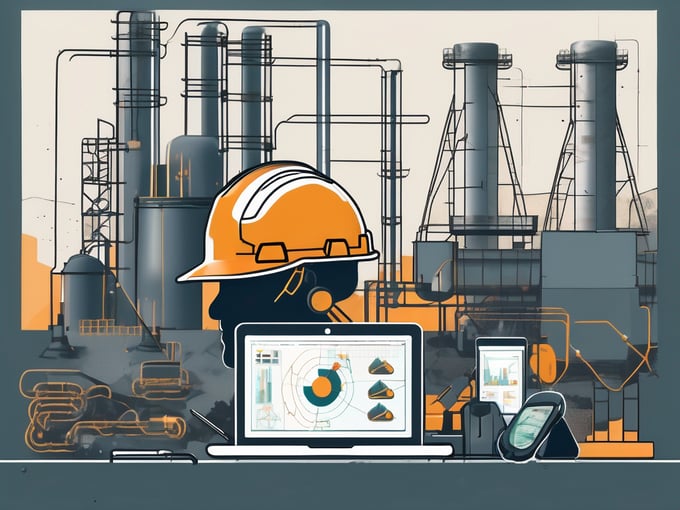
Important Trends in Data Analytics for Field Engineers in the Sludge Management Industry
Important Trends in Data Analytics for Field Engineers in the Sludge Management Industry
In the ever-evolving field of sludge management, data analytics has emerged as a crucial tool for field engineers. By harnessing the power of data, engineers can gain valuable insights into their operations, make informed decisions, and optimize efficiency. In this comprehensive guide, we will delve into the basics of data analytics, explore its significance in sludge management, discuss key concepts and techniques, and provide practical steps for implementation. Additionally, we will examine the role of data analytics in decision making and forecast the future of this rapidly advancing field.
Understanding the Basics of Data Analytics
Data analytics is the process of examining data sets to uncover patterns, draw conclusions, and make informed decisions. In the context of sludge management, it involves analyzing data generated throughout the lifecycle of sludge treatment, from collection and transportation to disposal. By leveraging various analytical tools and techniques, field engineers can extract valuable insights from this data, leading to enhanced operational efficiency and improved environmental outcomes.
When it comes to data analytics, there are several key concepts that field engineers need to understand. These concepts include descriptive, diagnostic, predictive, and prescriptive analytics. Descriptive analytics focuses on summarizing historical data to gain a better understanding of past events and trends. For example, field engineers can analyze historical data on sludge composition to identify any recurring patterns or changes over time.
Diagnostic analytics, on the other hand, involves examining data to uncover the causes behind certain outcomes or problems. In the context of sludge management, this could mean analyzing data on treatment efficiency to identify any factors that may be impacting the effectiveness of the treatment process. By understanding the root causes of problems, field engineers can then implement targeted solutions to improve overall performance.
Predictive analytics takes things a step further by using statistical models and machine learning algorithms to forecast future trends and scenarios. This can be incredibly valuable in the sludge management industry, as it allows field engineers to anticipate potential issues and take proactive measures to address them. For example, predictive analytics can help identify when equipment maintenance is likely to be needed, allowing for timely repairs and minimizing downtime.
Lastly, prescriptive analytics provides recommendations and optimization strategies based on the analysis of data. In the context of sludge management, this could involve using data analytics to identify the most efficient and cost-effective disposal methods, or to optimize resource allocation for transportation and treatment processes. By leveraging the power of data analytics, field engineers can make more informed decisions and drive continuous improvement in sludge management practices.
Importance of Data Analytics in Sludge Management
In the sludge management industry, data analytics plays a pivotal role in improving decision making, optimizing processes, and ensuring compliance with regulatory standards. By analyzing data on sludge composition, treatment efficiency, and operational parameters, field engineers can identify areas for improvement, optimize resource allocation, and reduce environmental impact.
One of the key benefits of data analytics in sludge management is the ability to identify areas for improvement. By analyzing data on treatment efficiency, field engineers can pinpoint any bottlenecks or inefficiencies in the process. This allows them to implement targeted solutions, such as optimizing equipment settings or adjusting treatment protocols, to improve overall performance and reduce costs.
Data analytics also enables proactive maintenance and predictive modeling in sludge management. By analyzing historical data on equipment performance and maintenance records, field engineers can identify patterns and trends that indicate when equipment is likely to fail or require maintenance. This allows for timely repairs or replacements, minimizing downtime and ensuring continuous operation of sludge treatment facilities.
Furthermore, data analytics in sludge management can help field engineers meet regulatory standards and ensure compliance. By analyzing data on sludge composition and environmental impact, engineers can ensure that treatment processes are meeting the required standards for disposal. This not only helps protect the environment but also avoids potential fines or penalties for non-compliance.
Key Data Analytics Concepts for Field Engineers
Field engineers working in the sludge management industry should be familiar with several important data analytics concepts. These concepts include data cleansing, data aggregation, data visualization, and data mining.
Data cleansing is the process of removing errors and inconsistencies from datasets. In the context of sludge management, this could involve identifying and correcting any inaccuracies in data on sludge composition or treatment efficiency. By ensuring the accuracy and reliability of the data, field engineers can make more confident decisions based on the insights derived from the analysis.
Data aggregation, on the other hand, involves summarizing and combining multiple data points. In sludge management, this could mean aggregating data from different treatment plants or collection sites to gain a broader perspective on overall performance. By combining data from various sources, field engineers can identify trends and patterns that may not be apparent when looking at individual datasets.
Data visualization is another important concept in data analytics. It allows for intuitive and effective communication of insights through charts, graphs, and maps. In the context of sludge management, data visualization can help field engineers communicate the results of their analysis to stakeholders, such as management or regulatory authorities. This visual representation of data can make it easier for non-technical audiences to understand and interpret the findings.
Lastly, data mining involves the discovery of patterns and relationships in large datasets using statistical techniques and machine learning algorithms. In the sludge management industry, data mining can be used to identify correlations between different variables, such as sludge composition and treatment efficiency. By uncovering these relationships, field engineers can gain valuable insights that can inform decision making and drive process optimization.
Tools and Techniques for Data Analytics in Sludge Management
To effectively utilize data analytics in the field of sludge management, engineers can leverage various tools and techniques. An overview of commonly used data analytics tools includes:
- Structured Query Language (SQL): SQL is a programming language specifically designed for managing and manipulating relational databases. It allows engineers to extract, transform, and analyze data efficiently.
- Statistical Software: Statistical software packages such as R and Python provide a wide range of statistical analysis and visualization capabilities. These tools enable engineers to perform complex analyses and generate visual representations of data.
- Data Visualization Tools: Tools like Tableau and Power BI facilitate the creation of interactive and visually appealing dashboards, allowing engineers to present insights in a user-friendly and intuitive manner.
In addition to these tools, several techniques are essential for successful data analytics in sludge management:
- Data Collection and Analysis Techniques: Field engineers must employ data collection techniques such as sampling and sensor deployment to ensure comprehensive data acquisition. They should then use data analysis techniques such as regression analysis, time series analysis, and clustering to gain insights and make informed decisions.
- Advanced Analytics Techniques: Advanced techniques such as machine learning, artificial intelligence, and predictive modeling provide engineers with the ability to forecast equipment failures, optimize maintenance schedules, and improve process efficiency. These techniques enable proactive decision making and yield significant cost savings.
When it comes to data collection techniques, engineers in the field of sludge management have to carefully consider the sampling methods they employ. Sampling is crucial as it allows them to gather representative data from different locations within a sludge management system. By strategically selecting sampling points, engineers can ensure that the collected data accurately reflects the overall condition of the system.
Furthermore, sensor deployment plays a vital role in data collection. Sensors can be strategically placed at various points in the sludge management system to continuously monitor important parameters such as temperature, pH levels, and flow rates. This real-time data can provide valuable insights into the system's performance and help identify any anomalies or potential issues.
Once the data is collected, engineers can apply various data analysis techniques to gain insights and make informed decisions. Regression analysis, for example, allows them to identify relationships between different variables and predict future trends. Time series analysis, on the other hand, helps in understanding patterns and detecting any seasonality or trends over time. Clustering techniques can be used to group similar data points together, enabling engineers to identify distinct patterns or anomalies within the dataset.
While data collection and analysis techniques are essential, advanced analytics techniques take sludge management to the next level. Machine learning algorithms, for instance, can be trained on historical data to predict equipment failures and optimize maintenance schedules. By analyzing patterns and identifying early warning signs, engineers can proactively address potential issues, minimizing downtime and reducing maintenance costs.
Artificial intelligence (AI) techniques can also be employed to automate decision-making processes in sludge management. AI algorithms can analyze vast amounts of data, identify patterns, and make recommendations for process optimization. This not only improves operational efficiency but also reduces the risk of human error.
Predictive modeling is another powerful technique that can be used in sludge management. By building models based on historical data, engineers can forecast future outcomes and make data-driven decisions. For example, predictive models can help optimize sludge treatment processes by determining the optimal dosage of chemicals or predicting the sludge's dewatering characteristics.
In conclusion, the combination of data analytics tools and techniques provides engineers in the field of sludge management with powerful capabilities to extract insights, optimize processes, and make informed decisions. By leveraging tools like SQL, statistical software, and data visualization tools, along with techniques such as data collection, regression analysis, and machine learning, engineers can improve the efficiency and effectiveness of sludge management systems, leading to cost savings and environmental sustainability.
Implementing Data Analytics in Field Engineering
Implementing data analytics in the field of sludge management requires careful planning and execution. The following steps can guide field engineers in this process:
- Define Objectives: Clearly articulate the goals and objectives of implementing data analytics. Identify the specific problems or challenges that need to be addressed and establish key performance indicators (KPIs) to measure success.
- Data Integration and Cleaning: Collect relevant data from various sources, ensuring data integrity and quality. Remove any inconsistencies or errors through thorough data cleansing and normalization.
- Analyze and Interpret: Utilize appropriate data analytics techniques to analyze the collected data. Look for patterns, anomalies, and correlations that can provide insights into operational performance and potential areas for improvement.
- Implement Solutions: Based on the insights gained from data analytics, develop and implement targeted solutions to address the identified issues. This may involve process optimization, equipment upgrades, or changes to operational procedures.
- Monitor and Measure: Continuously monitor the implemented solutions and measure their impact on key performance indicators. Collect feedback from field personnel and stakeholders to ensure the effectiveness of the implemented strategies.
Implementing data analytics in the field of sludge management can revolutionize the way engineers approach their work. By harnessing the power of data, field engineers can make informed decisions, optimize processes, and improve overall operational efficiency. The benefits of data analytics in this field are far-reaching, from reducing costs and minimizing environmental impact to enhancing the reliability and effectiveness of sludge management systems.
When defining objectives for data analytics implementation, it is crucial to involve key stakeholders and gain a comprehensive understanding of their needs and expectations. This collaborative approach ensures that the objectives are aligned with the organization's overall goals and that the implementation process is tailored to address specific challenges and requirements.
Data integration and cleaning is a critical step in the data analytics process. Field engineers must collect data from various sources, including sensors, monitoring systems, and historical records. This data may come in different formats and structures, making it essential to ensure data integrity and quality. Through thorough data cleansing and normalization, engineers can eliminate any inconsistencies or errors that may affect the accuracy and reliability of the analysis.
Once the data is collected and cleaned, field engineers can begin the analysis and interpretation phase. This is where the true power of data analytics comes into play. By applying appropriate techniques and algorithms, engineers can uncover valuable insights hidden within the data. They can identify patterns that indicate potential issues or opportunities for improvement, detect anomalies that require further investigation, and establish correlations between different variables that may impact operational performance.
Implementing solutions based on the insights gained from data analytics is a crucial step in the process. Field engineers can develop targeted strategies to address the identified issues and optimize sludge management processes. This may involve making changes to operational procedures, upgrading equipment, or implementing new technologies. By leveraging the power of data analytics, engineers can ensure that their solutions are data-driven, evidence-based, and tailored to the specific needs of the organization.
Monitoring and measuring the impact of the implemented solutions is essential to ensure their effectiveness. Field engineers must continuously monitor key performance indicators and collect feedback from field personnel and stakeholders. This feedback loop allows for ongoing evaluation and adjustment of the implemented strategies, ensuring that they deliver the desired outcomes and drive continuous improvement.
Overcoming Challenges in Data Analytics Implementation
Implementing data analytics in the field of sludge management may present some challenges. Limited data availability, data quality issues, and resistance to change are common obstacles. To overcome these challenges, field engineers should foster a data-driven culture within the organization, invest in data infrastructure and capabilities, and provide training and support to personnel involved in data analytics.
Limited data availability can be a significant challenge in data analytics implementation. Field engineers may struggle to gather sufficient data to perform meaningful analysis. In such cases, it is crucial to explore alternative data sources and consider implementing additional monitoring systems to collect the necessary data. Collaboration with external partners or industry experts can also provide access to relevant data sets and enhance the effectiveness of the analysis.
Data quality issues can undermine the accuracy and reliability of data analytics. Field engineers must ensure that the collected data is accurate, complete, and consistent. This may involve implementing data validation processes, conducting regular data audits, and investing in data quality management systems. By addressing data quality issues proactively, engineers can enhance the trustworthiness of the analysis and the resulting insights.
Resistance to change is another common challenge in data analytics implementation. Field engineers may encounter resistance from personnel who are accustomed to traditional methods and skeptical about the benefits of data analytics. To overcome this resistance, it is crucial to foster a data-driven culture within the organization. This involves educating and communicating the value of data analytics, providing training and support to personnel, and showcasing success stories and tangible outcomes resulting from data-driven decision-making.
Monitoring and Improving Data Analytics Processes
Continuous improvement is a crucial aspect of data analytics implementation. Field engineers should regularly evaluate the effectiveness of data analytics processes, identify areas for enhancement, and adopt emerging best practices. Collaborating with industry experts, attending conferences, and staying updated on the latest advancements in data analytics technology are all ways to ensure ongoing improvement.
Regular evaluation of data analytics processes allows field engineers to identify bottlenecks, inefficiencies, or areas where the analysis can be further optimized. By analyzing the entire data analytics workflow, engineers can streamline processes, eliminate redundant steps, and enhance the overall efficiency of the implementation. This continuous improvement mindset ensures that data analytics remains a dynamic and evolving discipline, capable of delivering maximum value to the field of sludge management.
Collaborating with industry experts and attending conferences is an excellent way for field engineers to stay updated on the latest advancements in data analytics technology. These events provide opportunities to learn from peers, gain insights from industry leaders, and discover new tools and techniques that can enhance the effectiveness of data analytics implementation. By staying at the forefront of technological advancements, field engineers can leverage the latest innovations to drive continuous improvement and stay ahead of the competition.
In conclusion, implementing data analytics in the field of sludge management is a complex process that requires careful planning, execution, and ongoing improvement. By following the steps outlined in this guide and overcoming the challenges associated with data analytics implementation, field engineers can unlock the full potential of data and revolutionize the way sludge management is approached. With data-driven decision-making, optimized processes, and continuous improvement, the field of sludge management can become more efficient, cost-effective, and environmentally sustainable.
Role of Data Analytics in Decision Making
Data-driven decision making has gained prominence in the sludge management industry. By utilizing data analytics, field engineers can make informed decisions based on empirical evidence rather than relying on intuition or guesswork.
Data-Driven Decision Making in Sludge Management
Data-driven decision making involves using data analysis to inform and guide decisions. In sludge management, field engineers can leverage data analytics to optimize treatment processes, allocate resources more efficiently, and reduce costs. By analyzing historical data and real-time operational data, engineers can identify trends, predict future scenarios, and make data-backed decisions that drive operational excellence.
Impact of Data Analytics on Operational Efficiency
The adoption of data analytics in sludge management has demonstrated significant improvements in operational efficiency. By analyzing data on operational performance, maintenance activities, and equipment health, engineers can identify bottlenecks, streamline processes, and reduce downtime. This leads to increased productivity, reduced costs, and enhanced overall operational performance.
Strategic Planning with Data Analytics
Data analytics enables field engineers to develop long-term strategic plans. By analyzing historical data and industry trends, engineers can forecast future demand, optimize capacity planning, and anticipate changes in regulations or market conditions. This strategic planning approach helps organizations stay ahead of the curve, adapt to evolving industry requirements, and maximize return on investment.
Future of Data Analytics in Sludge Management
The field of data analytics is continuously evolving, and its future in the sludge management industry is promising. As technology advances and new trends emerge, field engineers must prepare for the changes ahead.
Emerging Trends in Data Analytics
Data analytics is experiencing significant advancements in various areas. One emerging trend is the integration of Internet of Things (IoT) devices, which provide real-time data on equipment performance and operational parameters. This allows for better monitoring, predictive maintenance, and optimization of processes. Another trend is the use of artificial intelligence and machine learning algorithms to automate data analysis and generate actionable insights in real-time.
Predictive Analytics and Machine Learning in Sludge Management
Predictive analytics and machine learning techniques hold immense potential in sludge management. By leveraging historical and real-time data, engineers can develop predictive models that anticipate equipment failures, optimize maintenance schedules, and identify optimal treatment strategies. Machine learning algorithms can also enable autonomous decision making and continuous process optimization, leading to increased efficiency and reduced environmental impact.
Preparing for the Future of Data Analytics in Field Engineering
To leverage the future of data analytics in field engineering, field engineers should stay abreast of the latest advancements and continuously enhance their skills. Investing in training programs and collaborating with data scientists and experts in the field will help engineers adapt to the evolving landscape and harness the full potential of data analytics to drive innovation and success.