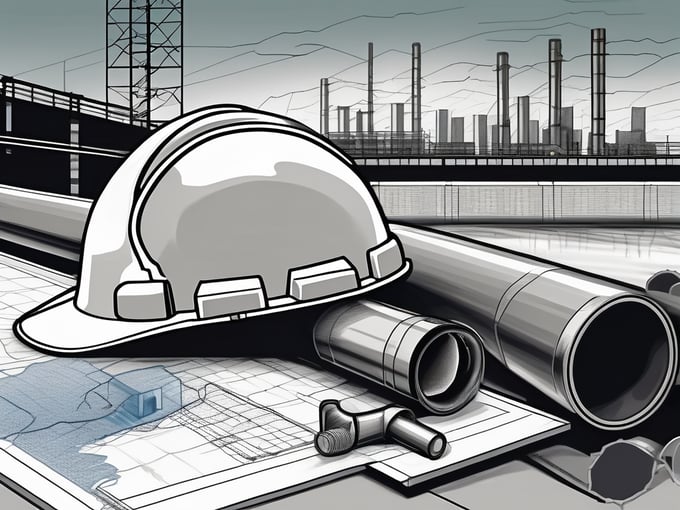
Important Trends in Data Analytics for Field Engineers in the Stormwater Industry
In today's rapidly advancing world, the stormwater industry is facing unprecedented challenges. The increasing frequency and intensity of storms, coupled with growing urbanization, have placed a significant strain on stormwater management systems. To address these challenges, field engineers are turning to data analytics as a powerful tool to improve their understanding of stormwater dynamics and make informed decisions.
Understanding the Importance of Data Analytics in the Stormwater Industry
Stormwater management is a complex field that requires a deep understanding of hydrologic processes, infrastructure design, and environmental regulations. Field engineers play a crucial role in ensuring the effective operation of stormwater systems and mitigating the risks associated with floods and water pollution.
Data analytics, often referred to as the science of examining raw data to uncover patterns and draw conclusions, has emerged as a game-changer in the stormwater industry. By harnessing the power of data, field engineers can gain valuable insights into the behavior of stormwater systems, identify areas of concern, and optimize their design and operation.
One of the key roles of field engineers in stormwater management is to design, construct, and maintain stormwater infrastructure. This includes drainage systems, retention ponds, and stormwater treatment facilities. These engineers are responsible for ensuring that these structures are built to withstand the forces of nature and effectively manage stormwater runoff.
With data analytics, field engineers can enhance their understanding of how stormwater systems perform in real-world conditions. By collecting and analyzing data from sensors, weather stations, and other monitoring devices, they can track water flows, pollutant levels, and system performance over time. This information enables them to identify potential issues, optimize system design, and improve the efficiency of stormwater management practices.
The Role of Field Engineers in Stormwater Management
Field engineers are responsible for designing, constructing, and maintaining stormwater infrastructure, such as drainage systems, retention ponds, and stormwater treatment facilities. They also conduct regular inspections and assessments to ensure compliance with regulatory standards.
With data analytics, field engineers can enhance their understanding of how stormwater systems perform in real-world conditions. By collecting and analyzing data from sensors, weather stations, and other monitoring devices, they can track water flows, pollutant levels, and system performance over time. This information enables them to identify potential issues, optimize system design, and improve the efficiency of stormwater management practices.
Field engineers also play a crucial role in mitigating the risks associated with stormwater runoff. They work closely with environmental scientists and regulatory agencies to ensure that stormwater management practices comply with local, state, and federal regulations. This includes implementing measures to prevent water pollution, such as the use of sediment basins and filtration systems.
In addition to their design and regulatory responsibilities, field engineers are also involved in the maintenance and operation of stormwater systems. They conduct regular inspections to identify any signs of damage or deterioration and take appropriate corrective actions. This may involve repairing or replacing damaged infrastructure, clearing debris from drainage systems, or conducting routine maintenance activities to ensure the optimal performance of stormwater systems.
The Impact of Data Analytics on the Stormwater Industry
The integration of data analytics into stormwater management strategies has revolutionized the industry. It has paved the way for evidence-based decision-making, resulting in more effective stormwater management practices and cost savings for municipalities and organizations.
Data analytics allows field engineers to identify trends, patterns, and anomalies in large datasets, providing valuable insights into stormwater dynamics. By correlating weather patterns with stormwater infrastructure performance, they can predict system vulnerabilities and optimize system design to withstand future events.
Furthermore, data analytics enables field engineers to accurately assess the effectiveness of stormwater management measures. By comparing pre- and post-implementation data, they can determine the success of their interventions and make adjustments if necessary.
Another significant impact of data analytics in the stormwater industry is the ability to monitor and manage stormwater systems in real-time. With the advent of advanced sensors and monitoring devices, field engineers can collect data on water levels, flow rates, and water quality parameters continuously. This real-time data allows them to respond quickly to changing conditions, such as heavy rainfall or increased pollutant levels, and take proactive measures to prevent flooding and water pollution.
In conclusion, data analytics has become an indispensable tool for field engineers in the stormwater industry. It empowers them to make informed decisions, optimize system design, and ensure the effective operation of stormwater infrastructure. By harnessing the power of data, field engineers can mitigate the risks associated with stormwater runoff, protect the environment, and create more resilient and sustainable stormwater management practices.
Fundamentals of Data Analytics
Before diving into the application of data analytics in field engineering, it is essential to understand the key concepts and tools involved.
Data analytics is a rapidly growing field that involves the extraction of insights and knowledge from large sets of data. It encompasses a wide range of techniques and methodologies, all aimed at making sense of the vast amount of information available in today's digital world.
Key concepts in data analytics include data preprocessing, exploratory data analysis, statistical modeling, and machine learning. Data preprocessing involves cleaning and transforming raw data to make it suitable for analysis. Exploratory data analysis focuses on understanding the data through visualizations and summary statistics. Statistical modeling allows for the identification of relationships and patterns in the data, while machine learning algorithms enable predictions and decision-making based on historical data.
Key Concepts in Data Analytics
Data preprocessing is a crucial step in the data analytics process. It involves cleaning and transforming raw data to ensure its quality and suitability for analysis. This may include removing duplicate or irrelevant data, handling missing values, and standardizing variables. By preprocessing the data, field engineers can ensure that their analysis is based on accurate and reliable information.
Exploratory data analysis is another important concept in data analytics. It involves the use of visualizations and summary statistics to gain insights into the data. Field engineers can explore the distribution of variables, identify outliers or anomalies, and detect patterns or trends. This exploratory phase helps in understanding the data and forming hypotheses for further analysis.
Statistical modeling is a powerful technique in data analytics. It allows field engineers to identify relationships and patterns in the data and make predictions or draw conclusions based on statistical inference. Statistical models can be used to test hypotheses, estimate parameters, and assess the significance of variables. By applying statistical modeling techniques, field engineers can gain a deeper understanding of the data and make informed decisions.
Machine learning is an advanced concept in data analytics that involves the use of algorithms to automatically learn from data and make predictions or decisions. Machine learning algorithms can be trained on historical data to recognize patterns and relationships, and then applied to new data to make predictions or classifications. Field engineers can use machine learning to automate tasks, optimize processes, and improve decision-making based on data-driven insights.
Tools and Techniques for Data Analysis
Various tools and techniques are available to field engineers for data analysis. These tools help in processing, analyzing, and visualizing data, making the task of extracting insights more efficient and effective.
Spreadsheets, such as Microsoft Excel or Google Sheets, are commonly used tools for data analysis. They provide a user-friendly interface for organizing and manipulating data, performing calculations, and creating visualizations. Spreadsheets are particularly useful for small to medium-sized datasets and simple analyses.
Statistical software, such as R or Python with libraries like Pandas or NumPy, offer more advanced capabilities for data analysis. These software packages provide a wide range of statistical functions, data manipulation tools, and visualization options. They are particularly useful for complex analyses, statistical modeling, and machine learning tasks.
Geographic Information Systems (GIS) are specialized tools for analyzing spatial data. They allow field engineers to visualize and analyze data in the context of geographic locations. GIS software enables the integration of various data sources, such as satellite imagery, maps, and demographic data, to gain insights into spatial patterns and relationships.
Cloud-based platforms, such as Amazon Web Services (AWS) or Google Cloud Platform (GCP), provide scalable and flexible solutions for data analysis. These platforms offer a wide range of tools and services, including data storage, processing, and analysis capabilities. Cloud-based platforms are particularly useful for handling large datasets, distributed computing, and collaborative analysis.
Each tool has its strengths and weaknesses, and the selection depends on the specific requirements of the analysis. Field engineers should consider factors such as data size, complexity, available resources, and desired outcomes when choosing the appropriate tools and techniques for their data analysis tasks.
Applying Data Analytics in Field Engineering
Now that we have covered the fundamentals of data analytics, let's explore how field engineers can effectively apply these techniques in their day-to-day work. By harnessing the power of data analytics, field engineers can gain valuable insights and make informed decisions to improve their operations and optimize their processes.
Data Collection and Management for Field Engineers
Data collection is a crucial step in the data analytics process for field engineers. To ensure accurate and reliable results, field engineers must carefully plan and execute their data collection efforts. This involves strategically placing sensors in key locations, setting up weather stations to monitor environmental conditions, and employing other monitoring techniques tailored to their specific field of work.
Field engineers must also focus on data management to effectively utilize the collected data. Proper data management practices include organizing data in a structured manner, cleaning and validating data to remove any inconsistencies or errors, and securely storing it for future analysis. By implementing robust data management protocols, field engineers can ensure the integrity of their analysis and facilitate collaboration with other stakeholders.
Furthermore, field engineers need to consider the scalability and sustainability of their data collection and management processes. As the volume of data continues to grow, field engineers must develop strategies to handle and process large datasets efficiently. This may involve leveraging cloud-based platforms or implementing automated data processing techniques to streamline their workflow.
Data Analysis and Interpretation in Field Engineering
Once the data is collected and managed, field engineers can proceed with data analysis. This phase involves applying appropriate statistical and machine learning techniques to uncover insights and patterns hidden within the data. By analyzing the collected data, field engineers can gain a deeper understanding of the systems they are working with and identify opportunities for improvement.
The analysis phase provides field engineers with a wealth of information. For example, in the field of hydrology, field engineers can analyze the relationships between rainfall events and runoff volumes to better understand the dynamics of water flow. They can also investigate the impact of land use changes on stormwater quality, helping them design more effective stormwater management strategies. Additionally, by analyzing the effectiveness of stormwater management interventions, field engineers can assess the success of their implemented measures and make data-driven decisions for future projects.
Interpreting the findings from data analysis is crucial for field engineers to make informed decisions and implement targeted strategies. By understanding the implications of their analysis, field engineers can develop innovative solutions to address challenges and improve the efficiency and sustainability of their operations. Effective interpretation of data analysis results also enables field engineers to communicate their findings to stakeholders, facilitating collaboration and driving positive change.
In conclusion, data analytics plays a vital role in field engineering. By collecting and managing data effectively, and analyzing and interpreting the results, field engineers can unlock valuable insights, optimize their processes, and make informed decisions to drive improvements in their field of work. Embracing data analytics empowers field engineers to tackle complex challenges and contribute to a more sustainable and efficient future.
Challenges and Solutions in Implementing Data Analytics
While data analytics offers tremendous potential in the field of stormwater engineering, there are several challenges that need to be addressed for successful implementation.
Data analytics has revolutionized the way field engineers approach problem-solving and decision-making. However, it is not without its obstacles. These challenges range from data availability and quality to integrating data analytics into existing workflows and decision-making processes.
Common Obstacles in Data Analytics for Field Engineers
One of the most common challenges in data analytics for field engineers is the availability and quality of data. Often, data may be incomplete, inconsistent, or subject to measurement errors. Field engineers must carefully assess the quality of the data and employ appropriate techniques to handle any limitations.
Furthermore, field engineers may encounter challenges in data collection. Gathering relevant data can be a time-consuming and resource-intensive process. It requires careful planning and coordination to ensure that all necessary data points are captured accurately and efficiently.
Another challenge is the integration of data analytics into existing workflows and decision-making processes. It may require a significant cultural shift and training to ensure that field engineers embrace data-driven approaches and effectively utilize the insights gained from data analytics. Resistance to change and a lack of understanding of the benefits of data analytics can hinder successful implementation.
Effective Strategies for Overcoming Data Analytics Challenges
To overcome these challenges, field engineers should establish a robust data governance framework that outlines data collection, management, and quality assurance protocols. This ensures that data is collected consistently and is of high quality. By implementing standardized procedures, field engineers can minimize errors and discrepancies in data, leading to more reliable and accurate analyses.
Training field engineers in data analytics techniques and providing them with access to appropriate tools and resources is crucial for successful implementation. Organizations should invest in ongoing professional development programs to enhance the data analytics skills of their field engineering teams. This can include training on data visualization, statistical analysis, and machine learning algorithms. By equipping field engineers with the necessary knowledge and tools, they can effectively leverage data analytics to make informed decisions and improve stormwater engineering practices.
In addition, fostering a culture of data-driven decision-making is essential. Organizations should encourage collaboration and knowledge sharing among field engineers, data scientists, and other stakeholders. This can be achieved through regular meetings, workshops, and conferences where best practices and success stories in data analytics are shared. By creating a supportive and collaborative environment, field engineers are more likely to embrace data analytics and incorporate it into their daily work.
Furthermore, organizations should consider partnering with external experts or consultants who specialize in data analytics for stormwater engineering. These experts can provide guidance and support in implementing data analytics initiatives, ensuring that field engineers have access to the latest methodologies and technologies.
In conclusion, while there are challenges in implementing data analytics in stormwater engineering, there are effective strategies to overcome them. By addressing data availability and quality, integrating data analytics into workflows, and investing in training and development, field engineers can harness the power of data analytics to drive innovation and improve decision-making in stormwater engineering.
Future Trends in Data Analytics for the Stormwater Industry
As technology continues to advance at a rapid pace, several future trends are set to shape the field of data analytics in the stormwater industry.
Emerging Technologies in Data Analytics
New technologies such as remote sensing, Internet of Things (IoT), and machine learning algorithms are revolutionizing the way data is collected, analyzed, and applied in field engineering. Remote sensing techniques, for example, enable field engineers to gather information about stormwater systems remotely, reducing the need for manual data collection.
Integrating IoT devices into stormwater infrastructure allows for real-time data monitoring and automated decision-making processes. Machine learning algorithms can analyze vast amounts of data to identify patterns and make predictions, supporting proactive maintenance and more efficient stormwater systems management.
The Future of Field Engineering with Data Analytics
Data analytics will continue to play a vital role in the future of field engineering in the stormwater industry. Advancements in technology will enable field engineers to collect, analyze, and interpret data more efficiently and accurately.
Furthermore, the integration of data analytics with other emerging technologies, such as artificial intelligence and blockchain, holds the potential to transform stormwater management practices. These technologies offer advanced data processing capabilities, improved decision-making, and enhanced transparency and accountability within the industry.
Conclusion
In conclusion, data analytics is transforming the field of stormwater engineering by providing field engineers with valuable insights into stormwater dynamics and enabling evidence-based decision-making. By harnessing the power of data, field engineers can enhance the design, operation, and maintenance of stormwater systems, ultimately leading to more effective stormwater management practices and resilient communities.