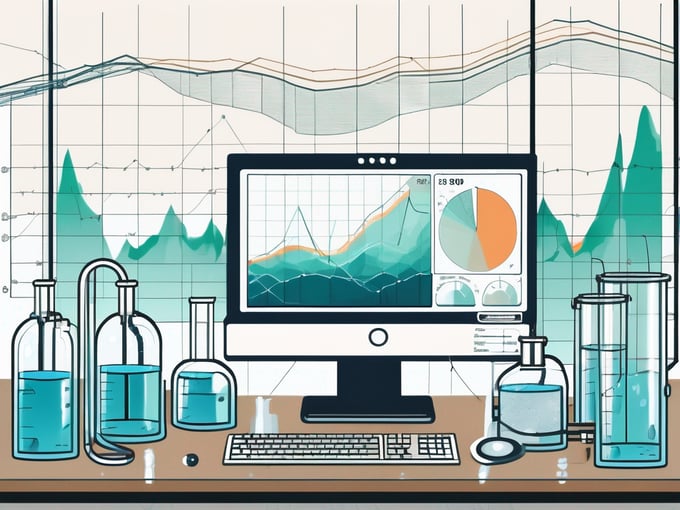
Important Trends in Data Analytics for Lab Analysts in the Groundwater Management Industry
In the world of groundwater management, data analytics has become an invaluable tool for lab analysts. With the ever-increasing complexity of groundwater systems and the need for accurate and timely information, the role of data analytics has never been more crucial. This ultimate guide aims to provide lab analysts with a comprehensive understanding of data analytics and how it can be applied in the groundwater management industry.
Understanding the Role of Data Analytics in Groundwater Management
Groundwater management relies heavily on the collection, analysis, and interpretation of data. Data analytics plays a vital role in this process by enabling lab analysts to make sense of the vast amounts of data they collect. By using various techniques and tools, analysts can uncover hidden patterns, trends, and correlations in the data, providing valuable insights that inform decision-making.
When it comes to groundwater management, the importance of data analytics cannot be overstated. It serves as a powerful tool that helps lab analysts and decision-makers understand the complex dynamics of groundwater systems. By analyzing data, they can gain a deeper understanding of the factors that influence groundwater quality, availability, and sustainability.
The Importance of Data Analytics in Groundwater Management
Data analytics is of paramount importance in groundwater management for several reasons. Firstly, it helps identify potential contamination sources and assess their impact on groundwater quality. By analyzing historical data, lab analysts can detect emerging trends and take proactive measures to mitigate pollution risks. For example, through data analytics, they can identify areas where industrial activities may be contributing to groundwater contamination and develop strategies to address the issue.
Secondly, data analytics enables accurate modeling of groundwater systems, allowing analysts to simulate various scenarios and develop effective management strategies. By incorporating data from multiple sources, such as groundwater level measurements, precipitation data, and land use information, analysts can create comprehensive models that reflect the complexities of the system. These models can then be used to evaluate the potential impacts of different management actions, such as implementing water conservation measures or establishing groundwater recharge programs.
Lastly, data analytics aids in predicting future trends and forecasting groundwater availability, helping decision-makers plan for sustainable water management. By analyzing historical data and using statistical techniques, analysts can identify long-term trends in groundwater levels and predict future changes. This information is crucial for developing strategies to ensure the long-term sustainability of groundwater resources, especially in regions facing water scarcity and increasing demand.
Key Data Analytics Concepts for Groundwater Management
In order to effectively harness the power of data analytics, lab analysts must grasp some key concepts. One important concept is data preprocessing, which involves cleaning, transforming, and integrating data from multiple sources. This ensures data accuracy and consistency, allowing for reliable analysis. For example, data preprocessing may involve removing outliers, filling in missing values, and standardizing units of measurement.
Another critical concept is statistical analysis, which aids in identifying patterns, trends, and anomalies in the data. Statistical techniques such as regression analysis and hypothesis testing provide valuable insights into the relationships between groundwater variables. For instance, regression analysis can help determine the relationship between groundwater levels and factors such as precipitation, land use, and pumping rates. Hypothesis testing, on the other hand, can be used to assess the significance of observed changes in groundwater quality over time.
Furthermore, data visualization is an essential aspect of data analytics in groundwater management. Visualizing data through charts, graphs, and maps allows analysts to communicate their findings effectively and facilitates better understanding among stakeholders. For instance, a map showing the spatial distribution of groundwater contamination can help decision-makers identify priority areas for remediation efforts.
In conclusion, data analytics plays a crucial role in groundwater management by providing valuable insights into groundwater quality, availability, and sustainability. By leveraging various techniques and concepts, lab analysts can analyze and interpret data to inform decision-making and develop effective management strategies. As the field of data analytics continues to advance, its potential for revolutionizing groundwater management grows, offering new opportunities for sustainable water resource management.
Essential Tools and Techniques for Data Analytics in Groundwater Management
To perform data analytics in groundwater management, lab analysts rely on a range of tools and techniques. One essential tool is Geographic Information System (GIS) software, which allows analysts to visualize and analyze spatial data. With GIS, analysts can map groundwater sources, contamination plumes, and other important features, aiding in decision-making processes. Additionally, data mining techniques are used to discover patterns and relationships within large datasets. These techniques include clustering, classification, and association rule mining, among others.
Data analytics in groundwater management involves a comprehensive approach that combines various tools and techniques to gain valuable insights. In addition to GIS software and data mining techniques, lab analysts also utilize other tools such as statistical software and specialized groundwater modeling software. These tools enable analysts to perform complex analyses, create visualizations, and simulate groundwater flow and contaminant transport.
One popular tool among lab analysts is R, a programming language and software environment for statistical analysis and graphics. R provides extensive libraries and packages specifically designed for data analysis in groundwater management. Analysts can use R to perform statistical tests, build predictive models, and generate interactive visualizations to communicate their findings effectively.
Python is another widely used tool in data analytics for groundwater management. It is a versatile programming language that offers a wide range of libraries and frameworks for data analysis, machine learning, and visualization. With Python, lab analysts can manipulate and analyze large datasets, apply advanced statistical techniques, and develop machine learning models to predict groundwater behavior and assess potential risks.
Another tool that lab analysts often rely on is MATLAB, a high-level programming language and environment for numerical computation, visualization, and programming. MATLAB provides a comprehensive set of functions and tools for data analysis, signal processing, and modeling. Analysts can use MATLAB to perform complex calculations, develop algorithms, and create interactive visualizations to gain deeper insights into groundwater dynamics.
Overview of Data Analytics Tools
There is a wide array of data analytics tools available for lab analysts in groundwater management. Some popular tools include R, Python, and MATLAB, which provide extensive libraries for data analysis and visualization. These tools allow analysts to conduct complex statistical analysis, create interactive visualizations, and build predictive models. Furthermore, specialized groundwater modeling software such as MODFLOW and FEFLOW assists in simulating groundwater flow and contaminant transport.
MODFLOW is a widely used groundwater modeling software that enables analysts to simulate and predict groundwater flow in various hydrogeological settings. It incorporates advanced numerical methods and algorithms to simulate the movement of groundwater through aquifers, considering factors such as recharge, discharge, and boundary conditions. MODFLOW also allows analysts to assess the impact of different management scenarios on groundwater resources and make informed decisions.
FEFLOW, on the other hand, is a comprehensive software package specifically designed for modeling groundwater flow and contaminant transport in complex hydrogeological systems. It incorporates advanced numerical methods and solvers to simulate the movement of groundwater and the transport of contaminants. FEFLOW also provides tools for uncertainty analysis and sensitivity analysis, allowing analysts to assess the reliability of their models and evaluate the potential impacts of uncertainties.
Techniques for Analyzing Groundwater Data
Lab analysts employ various techniques to analyze groundwater data effectively. One such technique is time series analysis, which examines the temporal variations of groundwater variables. This analysis helps identify seasonal patterns, long-term trends, and short-term fluctuations in groundwater levels and quality. By analyzing time series data, analysts can detect changes in groundwater dynamics over time and assess the impact of external factors such as climate change and human activities.
Spatial analysis is another important technique used in groundwater data analysis. It involves investigating the spatial distribution and relationships of groundwater parameters such as water levels, quality, and contaminant concentrations. Spatial analysis helps identify potential contamination sources, understand the spatial variability of groundwater properties, and plan effective groundwater monitoring networks. By combining spatial analysis with GIS software, analysts can create maps and spatial models that provide valuable insights into groundwater management strategies.
In addition to time series analysis and spatial analysis, lab analysts also utilize statistical analysis techniques to gain a deeper understanding of groundwater data. Statistical analysis involves applying various statistical tests and models to analyze the relationships between different variables, assess the significance of trends and patterns, and make predictions about future groundwater behavior. By employing statistical techniques such as regression analysis, hypothesis testing, and multivariate analysis, analysts can uncover hidden patterns and correlations in groundwater data and make evidence-based decisions.
The Process of Data Collection in Groundwater Management
Data collection is a crucial step in groundwater management, as it serves as the foundation for all subsequent analysis and interpretation. Lab analysts must follow best practices to ensure accurate and reliable data collection.
Groundwater management involves the monitoring and assessment of water resources beneath the Earth's surface. It is essential for understanding the quantity and quality of groundwater, which is vital for various purposes such as drinking water supply, agriculture, and industrial use. To effectively manage groundwater resources, data collection plays a pivotal role.
Best Practices for Data Collection
When collecting groundwater data, lab analysts should adhere to several best practices. Firstly, data collection should be conducted using standardized protocols, ensuring consistency across different sampling locations and time periods. Standardization allows for reliable comparisons and trend analysis, enabling scientists and policymakers to make informed decisions.
Secondly, analysts must properly calibrate and maintain monitoring equipment to ensure accurate measurements. Regular equipment calibration and maintenance schedules should be implemented to minimize measurement errors. This includes checking for instrument drift, which can lead to inaccurate readings over time. By regularly calibrating instruments, analysts can ensure the reliability of collected data.
Additionally, adequate sample sizes should be collected to ensure statistical validity. The number of samples collected should be determined based on the variability of groundwater characteristics and the desired level of confidence in the data. Collecting a sufficient number of samples reduces the risk of sampling bias and provides a more representative picture of the groundwater system.
Finally, data should be recorded in a reliable database system that facilitates easy retrieval and analysis. A well-designed database system allows for efficient data management, ensuring that collected information is organized, accessible, and can be easily integrated with other datasets. This enables scientists and water resource managers to conduct comprehensive analyses and develop effective groundwater management strategies.
Common Challenges in Data Collection and How to Overcome Them
Data collection in groundwater management often faces numerous challenges, which can impact data quality and reliability. One common challenge is the presence of measurement errors, which can arise from factors such as instrument drift or human error. To mitigate this challenge, analysts should regularly calibrate instruments and conduct quality control checks during data collection. By implementing rigorous quality control measures, analysts can identify and correct any measurement errors, ensuring the accuracy of the collected data.
Another challenge is the spatial variability of groundwater, which can lead to biased sampling. Groundwater characteristics can vary significantly over short distances, making it crucial to design monitoring networks that capture representative groundwater conditions. This involves strategically selecting monitoring well locations and considering factors such as hydrogeological settings, land use patterns, and potential contaminant sources. By carefully designing monitoring networks, analysts can minimize sampling bias and obtain a more comprehensive understanding of the groundwater system.
Furthermore, data collection in groundwater management often involves working in remote and challenging environments. Fieldwork can be physically demanding, requiring analysts to navigate rugged terrains and adverse weather conditions. It is essential for analysts to prioritize safety and adhere to proper fieldwork protocols. This includes wearing appropriate personal protective equipment, conducting risk assessments, and following established safety guidelines.
In conclusion, data collection is a critical component of groundwater management. By following best practices and overcoming common challenges, lab analysts can ensure the accuracy, reliability, and representativeness of collected data. This, in turn, enables informed decision-making and effective management of groundwater resources for the benefit of present and future generations.
Data Analysis and Interpretation in Groundwater Management
Data analysis and interpretation are crucial steps in groundwater management, as they transform raw data into meaningful insights. Lab analysts must follow a systematic approach to ensure accurate analysis and interpretation.
Steps in Data Analysis
Data analysis in groundwater management typically involves several key steps. Firstly, analysts must explore and visualize the data to identify any outliers or data quality issues. This step helps ensure data integrity before proceeding to the next steps. Secondly, analysts apply appropriate statistical techniques to analyze the data, such as regression analysis or time series analysis. These techniques provide valuable information on relationships between groundwater variables and temporal trends. Lastly, analysts interpret the results of the analysis, drawing meaningful conclusions and making recommendations based on the data.
Tips for Accurate Data Interpretation
Accurate data interpretation is essential to derive meaningful insights and inform decision-making. Lab analysts should consider the following tips for accurate interpretation. Firstly, analysts should clearly define the objectives and research questions before interpreting the data. This ensures that the interpretation aligns with the desired outcomes. Secondly, analysts should consider the context in which the data was collected and the specific characteristics of the groundwater system being studied. This context helps to ensure that interpretations are relevant and applicable. Lastly, analysts should validate their interpretations by considering multiple lines of evidence and seeking input from domain experts.
Application of Data Analytics in Groundwater Management
Data analytics has revolutionized the field of groundwater management, providing lab analysts with powerful tools to address complex challenges.
Predictive Analytics in Groundwater Management
Predictive analytics leverages historical data and statistical modeling techniques to forecast future trends in groundwater systems. Lab analysts can use predictive analytics to estimate future groundwater levels, identify potential contamination hotspots, and simulate the impacts of climate change scenarios. This proactive approach enables decision-makers to implement preventive measures and develop sustainable long-term strategies.
Data-Driven Decision Making in Groundwater Management
Data analytics enables data-driven decision making by providing evidence-based insights. Lab analysts can analyze large datasets to identify trends, patterns, and potential risks, supporting more informed and effective decision-making processes. Whether it is developing groundwater management plans, setting regulatory thresholds, or optimizing monitoring networks, data analytics plays a crucial role in guiding decision-makers.
Future Trends in Data Analytics for Groundwater Management
The field of data analytics is continually evolving, driven by advancements in technology and increasing data availability. Several future trends are poised to shape the landscape of groundwater management.
The Role of Artificial Intelligence in Groundwater Data Analytics
Artificial Intelligence (AI) techniques, such as machine learning and neural networks, hold great potential in groundwater data analytics. These techniques can identify complex patterns and relationships in large datasets that may not be readily apparent through traditional analysis methods. AI can also assist in optimizing groundwater management strategies by continuously learning from real-time data and providing adaptive recommendations.
The Impact of Big Data on Groundwater Management
With the advent of big data, the volume, velocity, and variety of groundwater data have increased dramatically. This presents both challenges and opportunities for lab analysts in groundwater management. Harnessing big data requires advanced data storage, processing, and analysis techniques. However, big data also offers the potential for enhanced understanding of groundwater systems through the integration of diverse datasets and the application of advanced analytics.
As lab analysts in the groundwater management industry, understanding and harnessing the power of data analytics is crucial. By utilizing the tools, techniques, and concepts discussed in this guide, lab analysts can make informed decisions, develop effective strategies, and contribute to the sustainable management of groundwater resources.