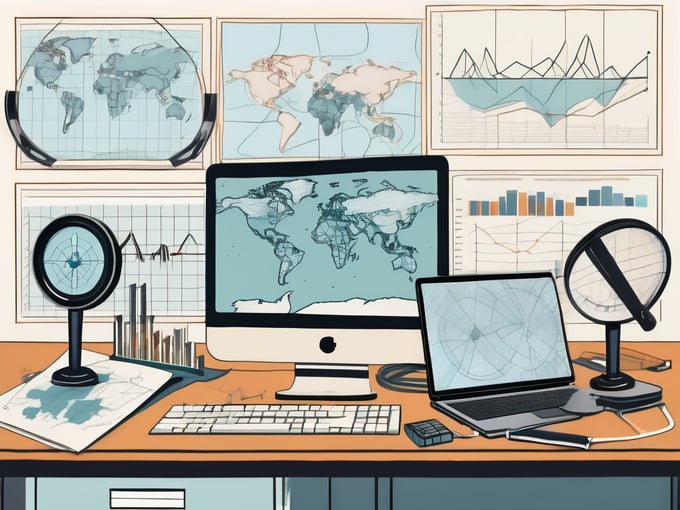
Important Trends in Data Analytics for Superintendents in the Groundwater Management Industry
In the rapidly evolving field of groundwater management, the ability to harness data analytics has become increasingly crucial. As a superintendent in the groundwater management industry, understanding the importance of data analytics and how to implement it effectively can greatly enhance your decision-making processes and improve overall efficiency. This comprehensive guide will walk you through the key concepts, implementation strategies, and future trends of data analytics in groundwater management.
Understanding the Importance of Data Analytics in Groundwater Management
As a superintendent, it is essential to recognize the pivotal role that data analytics plays in groundwater management. By leveraging data analytics tools and techniques, you can gain valuable insights into the behavior and trends of groundwater resources. This knowledge empowers you to make informed decisions, implement proactive strategies, and optimize resource allocation within your management system.
The Role of Superintendents in Groundwater Management
Superintendents are responsible for overseeing the operations and maintenance of groundwater management systems. They play a crucial role in ensuring the sustainable use of groundwater resources and mitigating potential risks. By utilizing data analytics, you can monitor and analyze key performance indicators, evaluate the effectiveness of different management practices, and identify opportunities for improvement.
For example, through data analytics, superintendents can track the water levels in various wells and assess the impact of pumping activities on the overall groundwater system. They can also analyze historical data to identify patterns and trends, enabling them to anticipate potential issues and take proactive measures to address them. By leveraging data-driven decision-making, superintendents can enhance the sustainability and long-term viability of groundwater resources.
The Intersection of Data Analytics and Groundwater Management
Data analytics provides a powerful framework for understanding the complex interactions between geological, hydrological, and environmental factors that influence groundwater dynamics. By integrating data from various sources, such as monitoring wells, satellite remote sensing, and climatic databases, you can develop comprehensive models that accurately represent the behavior of your groundwater system.
For instance, data analytics can help identify the factors contributing to groundwater contamination, such as industrial activities or agricultural practices. By analyzing the spatial and temporal patterns of contamination, superintendents can implement targeted remediation strategies to protect the quality of groundwater resources.
Data analytics also enables superintendents to assess the impact of climate change on groundwater availability. By analyzing long-term climate data and groundwater level measurements, they can identify trends and patterns that indicate potential changes in recharge rates or aquifer storage capacity. This information is crucial for developing adaptive management strategies that ensure the sustainable use of groundwater resources in the face of changing environmental conditions.
Furthermore, data analytics can facilitate the integration of groundwater management with other water resource management systems. By analyzing data from surface water sources, such as rivers and lakes, superintendents can assess the interactions between surface water and groundwater, enabling them to make informed decisions regarding water allocation and resource optimization.
In conclusion, data analytics plays a vital role in groundwater management, empowering superintendents to make informed decisions, optimize resource allocation, and ensure the long-term sustainability of groundwater resources. By leveraging data analytics tools and techniques, superintendents can gain valuable insights into groundwater behavior and trends, evaluate the effectiveness of management practices, and develop comprehensive models that accurately represent the dynamics of the groundwater system. The integration of data analytics with groundwater management enhances the understanding of complex interactions, enables targeted remediation strategies, assesses the impact of climate change, and facilitates the integration of surface water and groundwater management systems.
Key Concepts in Data Analytics
To effectively implement data analytics in groundwater management, it is essential to grasp the fundamental concepts and techniques. Two key aspects of data analytics in this context are data collection and processing, and data interpretation and visualization.
Data Collection and Processing
Data collection involves gathering relevant information about your groundwater system through various means, such as field measurements, laboratory analysis, and remote sensing technologies. Field measurements provide real-time data on groundwater levels, flow rates, and quality parameters. This data is collected using specialized instruments and equipment, such as groundwater level loggers, flow meters, and water quality sensors.
Laboratory analysis plays a crucial role in data collection, as it allows for the detailed examination of water samples. Various parameters, including pH, conductivity, dissolved oxygen, and the presence of contaminants, can be measured in the laboratory. These analyses provide valuable insights into the chemical composition and overall health of the groundwater system.
In recent years, remote sensing technologies have revolutionized data collection in groundwater management. Satellite imagery, aerial photography, and LiDAR (Light Detection and Ranging) data can be used to assess land cover, identify potential pollution sources, and monitor changes in groundwater levels over time. These remote sensing techniques provide a broader perspective and complement the data collected through field measurements and laboratory analysis.
Once collected, this data needs to be processed to remove any inconsistencies, errors, or outliers that may impact the accuracy of subsequent analyses. Sophisticated algorithms and statistical techniques are employed to ensure data quality and integrity. Data validation and quality control measures are implemented to identify and correct any errors or inconsistencies in the dataset. Outliers, which are data points that deviate significantly from the expected pattern, are carefully examined to determine their validity and potential impact on the analysis.
Data processing also involves data integration and transformation. Different datasets, such as groundwater levels, rainfall data, and land use information, are combined to gain a comprehensive understanding of the groundwater system. Data transformation techniques, such as normalization and aggregation, are applied to standardize and summarize the data, making it suitable for further analysis.
Data Interpretation and Visualization
Data interpretation involves extracting meaningful insights from raw data to facilitate decision-making processes. By utilizing statistical models, machine learning algorithms, and trend analysis, you can identify patterns, anomalies, and correlations within your dataset. Statistical models, such as regression analysis and time series analysis, can be used to establish relationships between different variables and predict future groundwater conditions.
Machine learning algorithms, including clustering, classification, and anomaly detection, can uncover hidden patterns and anomalies in the data. These algorithms learn from the data and can automatically identify complex relationships and outliers that may not be apparent through traditional statistical methods.
Trend analysis is another important technique in data interpretation. By analyzing historical data, you can identify long-term trends and patterns, such as seasonal variations or gradual changes in groundwater levels. This information can help in understanding the underlying processes and making informed decisions.
These insights can then be visualized using powerful graphical tools, such as charts, graphs, and maps, to enhance understanding and facilitate effective communication. Line charts and scatter plots can be used to visualize temporal trends and relationships between variables. Heatmaps and contour maps can provide a spatial representation of groundwater levels and quality across the study area.
Interactive visualization tools, such as dashboards and web-based applications, allow stakeholders to explore the data and gain insights in a user-friendly manner. These tools enable decision-makers to interact with the data, apply filters, and view different scenarios, empowering them to make informed decisions based on the analysis results.
In conclusion, data analytics in groundwater management involves the collection, processing, interpretation, and visualization of data. Through these key concepts, stakeholders can gain valuable insights into the groundwater system, identify potential issues, and make informed decisions to ensure the sustainable management of this vital resource.
Implementing Data Analytics in Groundwater Management
Implementing data analytics in groundwater management requires careful consideration of various factors, including choosing the right data analytics tools and overcoming implementation challenges.
Groundwater management plays a crucial role in ensuring the sustainability and availability of this vital natural resource. With the increasing complexity of groundwater systems and the growing demand for water, data analytics has emerged as a valuable tool for understanding and managing groundwater resources effectively.
Choosing the Right Data Analytics Tools
There is a wide range of data analytics tools available, each with its own strengths and limitations. It is essential to evaluate your specific needs and goals before selecting a tool. Factors to consider include the ease of use, scalability, compatibility with existing systems, and the availability of technical support.
One popular data analytics tool is machine learning, which uses algorithms to analyze large datasets and identify patterns and trends. Machine learning algorithms can help predict groundwater levels, detect anomalies, and optimize pumping strategies. Another tool is geographic information systems (GIS), which allows for the visualization and analysis of spatial data, helping groundwater managers make informed decisions.
Working in collaboration with data scientists and IT professionals can help ensure the successful implementation of the chosen tool. Their expertise can assist in data preprocessing, model development, and result interpretation. Furthermore, involving stakeholders, such as water resource managers and local communities, in the decision-making process can provide valuable insights and enhance the effectiveness of data analytics in groundwater management.
Overcoming Challenges in Data Implementation
Implementing data analytics in groundwater management may come with various challenges, such as data availability, data quality, and data integration. Ensuring that data is collected consistently and stored in a standardized format is vital. This requires establishing robust data collection protocols, including the use of reliable sensors and data loggers.
Data quality is another critical aspect to consider. Inaccurate or incomplete data can lead to erroneous conclusions and ineffective management strategies. Implementing quality control measures, such as data validation and verification processes, can help ensure the accuracy and reliability of the collected data.
Data integration is often a challenge in groundwater management, as data is collected from multiple sources and in different formats. Developing data integration frameworks, such as data warehouses or data lakes, can facilitate the aggregation and harmonization of diverse datasets. This allows for a comprehensive analysis of groundwater resources and enables informed decision-making.
Additionally, developing strong data governance protocols, including data security and privacy measures, is essential to maintain the integrity and confidentiality of sensitive information. Ensuring compliance with data protection regulations and implementing access controls can help safeguard data from unauthorized access or misuse.
In conclusion, implementing data analytics in groundwater management requires careful consideration of various factors. Choosing the right data analytics tools and overcoming implementation challenges are crucial steps towards effectively managing groundwater resources. By leveraging the power of data analytics, groundwater managers can gain valuable insights, make informed decisions, and ensure the sustainable use of this vital natural resource.
Leveraging Data Analytics for Decision Making
One of the key benefits of data analytics is its ability to provide predictive insights that enable proactive decision-making in groundwater management.
Data analytics has revolutionized the way we approach groundwater management. By harnessing the power of historical data and applying advanced modeling techniques, predictive analytics can help you anticipate future changes in groundwater levels, quality, and availability. This empowers you to proactively plan for potential challenges and implement appropriate management strategies in a timely manner.
Imagine being able to predict the future behavior of groundwater resources with a high level of accuracy. With data analytics, this is now possible. By analyzing vast amounts of data collected over time, you can identify patterns and trends that can help you make informed decisions about groundwater management.
Predictive Analytics for Proactive Management
By leveraging historical data and applying advanced modeling techniques, predictive analytics can help you anticipate future changes in groundwater levels, quality, and availability. This empowers you to proactively plan for potential challenges and implement appropriate management strategies in a timely manner.
For example, let's say you are responsible for managing a groundwater basin that supplies water to a growing population. By analyzing historical data on groundwater levels, rainfall patterns, and population growth, data analytics can help you predict future water demand and plan for infrastructure upgrades or alternative water sources well in advance. This proactive approach ensures that water supply meets the needs of the population without any disruptions.
Predictive analytics also plays a crucial role in managing water scarcity. By analyzing data on climate patterns, water usage, and groundwater levels, you can identify areas that are at risk of water scarcity in the future. This allows you to implement conservation measures, such as water restrictions or alternative water sources, to mitigate the impact of water scarcity on communities and ecosystems.
Data-Driven Strategies for Efficient Groundwater Management
Data analytics enables you to identify inefficiencies and optimize resource allocation within your groundwater management system. By analyzing data on extraction rates, recharge rates, and other key metrics, you can develop data-driven strategies that maximize water availability while minimizing environmental impact and operational costs.
For instance, data analytics can help you identify areas where groundwater extraction is exceeding sustainable levels. By analyzing extraction rates and recharge rates, you can determine the optimal extraction limits for each area to ensure long-term sustainability. This data-driven approach not only protects the groundwater resources but also ensures that water is allocated efficiently to meet the needs of various stakeholders.
Data analytics also allows you to optimize the allocation of resources within your groundwater management system. By analyzing data on water quality, usage patterns, and infrastructure efficiency, you can identify areas where improvements can be made. This could involve upgrading infrastructure, implementing water treatment technologies, or optimizing distribution networks to minimize water loss.
In conclusion, data analytics has transformed groundwater management by providing predictive insights and enabling data-driven decision-making. By leveraging historical data and advanced modeling techniques, you can anticipate future changes, plan for potential challenges, and optimize resource allocation. This proactive and efficient approach ensures the sustainable management of groundwater resources for the benefit of present and future generations.
Ensuring Data Security and Compliance
With the increasing reliance on data analytics in groundwater management, it is essential to prioritize data security and comply with relevant privacy laws and regulations.
Understanding Data Privacy Laws and Regulations
It is crucial to be aware of the legal and ethical implications surrounding the collection, storage, and use of data in groundwater management. Several jurisdictions have enacted legislation to protect individual privacy and ensure responsible data handling. Familiarize yourself with these laws and establish robust protocols to maintain compliance.
Best Practices for Data Security in Groundwater Management
To safeguard sensitive data, it is important to implement best practices for data security. This includes using secure storage and transmission methods, encrypting data at rest and in transit, regularly updating and patching software, and implementing access controls and user authentication protocols. Regular monitoring and auditing of data access and usage should also be conducted to detect and prevent any potential breaches.
The Future of Data Analytics in Groundwater Management
Data analytics is an ever-evolving field, and staying abreast of emerging trends and technologies can give you a competitive edge in groundwater management.
Emerging Trends in Data Analytics
Advances in machine learning, artificial intelligence, and big data analytics are revolutionizing the way data is processed, analyzed, and utilized in groundwater management. Keeping abreast of these emerging trends can help you harness the full potential of data analytics to enhance your decision-making processes.
Preparing for the Future of Groundwater Management
As the demand for sustainable water management practices grows, so does the importance of data analytics in groundwater management. By continuously updating your knowledge and skills in data analytics, you can position yourself as a leader in the field and contribute to the long-term sustainability of groundwater resources.
By understanding the importance of data analytics, harnessing its power through implementation, and staying ahead of emerging trends, you can become a proactive superintendent in the groundwater management industry. The insights gained through data analytics will not only optimize operational efficiency but also ensure the long-term sustainability of groundwater resources for future generations.