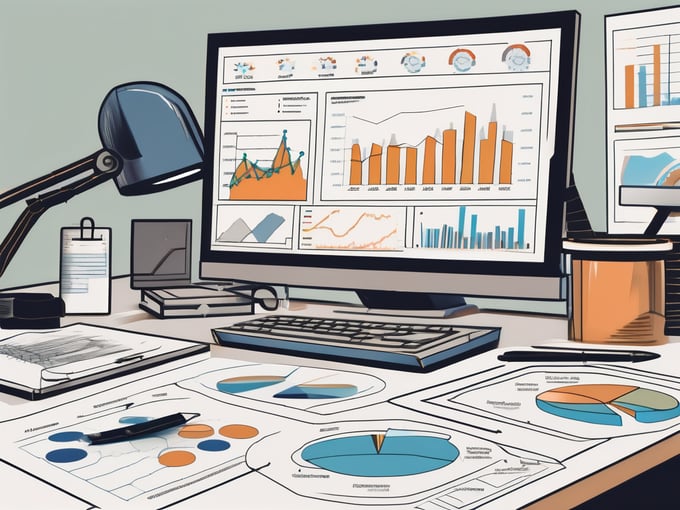
Important Trends in Data Analytics for Superintendents in the Sludge Management Industry
In the sludge management industry, the effective use of data analytics can make a significant impact on operational efficiency, cost reduction, and environmental sustainability. Superintendents play a critical role in harnessing the power of data to drive informed decision-making and improve overall performance. This ultimate guide will provide you with a comprehensive understanding of data analytics in sludge management and equip you with practical knowledge to implement and utilize data analytics effectively.
Understanding the Importance of Data Analytics in Sludge Management
Sludge management involves the treatment and disposal of wastewater residuals, a crucial process in maintaining public health and environmental well-being. By leveraging data analytics, superintendents can gain valuable insights into operational processes, performance benchmarks, and potential areas for improvement. Such insights allow for proactive decision-making, leading to optimized resource allocation, enhanced system reliability, and improved regulatory compliance.
As a superintendent, your role in data analysis is vital. By understanding the fundamental concepts and benefits of data analytics, you can drive positive change within your organization and contribute to the advancement of the sludge management industry as a whole.
Data analytics in sludge management goes beyond basic data collection and reporting. It involves the use of advanced statistical techniques and algorithms to analyze large datasets, uncover patterns, and identify correlations. These analytical tools enable superintendents to make data-driven decisions, rather than relying solely on intuition or past experiences.
One of the key benefits of data analytics in sludge management is the ability to optimize resource allocation. By analyzing historical data on sludge production, treatment processes, and disposal methods, superintendents can identify areas where resources are being underutilized or misallocated. This allows for more efficient resource allocation, reducing costs and improving overall operational performance.
In addition to resource optimization, data analytics can also enhance system reliability. By monitoring key performance indicators (KPIs) such as sludge dewatering efficiency, solids content, and treatment process effectiveness, superintendents can identify potential issues before they escalate into major problems. This proactive approach to system maintenance minimizes downtime, reduces the risk of equipment failure, and ensures consistent and reliable sludge management operations.
Furthermore, data analytics can help superintendents improve regulatory compliance. By analyzing historical data on effluent quality, discharge limits, and regulatory requirements, superintendents can identify areas where compliance may be at risk. This allows for timely corrective actions to be taken, ensuring that the organization meets all regulatory obligations and avoids costly penalties or reputational damage.
As the superintendent, your understanding of data analytics can also contribute to the advancement of the sludge management industry as a whole. By sharing best practices, lessons learned, and success stories with other professionals in the field, you can help drive innovation and continuous improvement. Collaboration and knowledge sharing are key to addressing the challenges and complexities of sludge management in an ever-changing regulatory and environmental landscape.
In conclusion, data analytics plays a crucial role in sludge management by providing valuable insights, optimizing resource allocation, enhancing system reliability, and improving regulatory compliance. As a superintendent, your understanding of data analytics can drive positive change within your organization and contribute to the advancement of the sludge management industry. Embracing data analytics as a superintendent is not just about analyzing numbers, but about leveraging insights to make informed decisions and improve overall operational performance.
Key Benefits of Applying Data Analytics in Sludge Management
Implementing data analytics in sludge management offers numerous benefits. Firstly, it allows for the identification of patterns and trends in data, offering insights into system performance and potential operational issues. This empowers superintendents to make data-driven decisions that streamline processes, reduce costs, and improve overall efficiency.
For example, by analyzing data on sludge composition and characteristics, data analytics can help identify the most effective treatment methods and optimize the use of chemicals and resources. This not only improves the quality of the treated sludge but also reduces the environmental impact of the treatment process.
Secondly, data analytics enables the prediction of maintenance needs and equipment failures. By utilizing predictive analytics models, superintendents can proactively address maintenance requirements, preventing costly breakdowns and minimizing system downtime.
Imagine a scenario where data analytics detects a potential equipment failure in the sludge dewatering process. By analyzing historical data and identifying patterns that precede equipment failures, the system can automatically generate maintenance alerts, allowing the maintenance team to take immediate action and prevent a major breakdown. This predictive approach not only saves time and money but also ensures uninterrupted sludge management operations.
Furthermore, data analytics facilitates the identification of energy-saving opportunities, helping superintendents optimize energy usage and reduce operational expenses. Through detailed analysis of energy consumption data, opportunities for energy efficiency improvements can be identified and implemented, resulting in significant cost savings.
For instance, data analytics can analyze energy consumption patterns in different sludge treatment stages and identify areas of high energy consumption. By implementing energy-saving measures such as optimizing aeration processes or utilizing renewable energy sources, superintendents can reduce energy costs and minimize the environmental impact of sludge management.
In addition to these benefits, data analytics can also enhance regulatory compliance in sludge management. By analyzing data on sludge composition and treatment processes, superintendents can ensure that the sludge meets regulatory standards and avoid potential fines or penalties.
Moreover, data analytics can improve the overall transparency and accountability of sludge management operations. By collecting and analyzing data on sludge quantities, treatment processes, and disposal methods, superintendents can provide detailed reports and evidence of compliance to stakeholders, regulatory agencies, and the public.
In conclusion, the application of data analytics in sludge management offers a wide range of benefits. From optimizing processes and reducing costs to improving energy efficiency and ensuring regulatory compliance, data analytics empowers superintendents to make informed decisions and drive continuous improvement in sludge management operations.
Fundamentals of Data Analytics
Basic Concepts and Terminology
Before diving into the practical aspects of data analytics, it is essential to grasp the basic concepts and terminology. Data analytics involves the collection, transformation, analysis, and interpretation of data to extract meaningful insights. This field has gained significant importance in recent years due to the increasing availability of data and the need for data-driven decision-making.
Key concepts in data analytics include data aggregation, data mining, statistical analysis, and visualization techniques. Data aggregation involves the collection and organization of data from various sources, allowing for comprehensive analysis. By combining data from multiple sources, analysts can gain a holistic view of the subject matter and identify patterns and trends that may not be apparent when looking at individual datasets.
Data mining refers to the process of discovering patterns and correlations within large datasets. It involves using advanced algorithms and statistical techniques to uncover hidden insights and relationships. This process can be particularly useful in identifying customer behavior patterns, market trends, and anomalies in data.
Statistical analysis is a fundamental component of data analytics. It enables quantifiable conclusions to be drawn from data, providing a solid foundation for decision-making. By applying statistical techniques, analysts can identify significant relationships, test hypotheses, and make predictions based on data patterns.
Visualization techniques play a crucial role in data analytics as they facilitate the clear presentation of complex information. By representing data visually through charts, graphs, and interactive dashboards, analysts can communicate their findings effectively. Visualization tools, such as Tableau and Power BI, allow for the creation of interactive visualizations that enable users to explore data and gain insights intuitively.
Tools and Techniques for Data Analysis
There are various tools and techniques available to superintendents for effective data analysis. Statistical software packages, such as R and Python, provide robust capabilities for data manipulation, visualization, and analysis. These programming languages offer a wide range of libraries and packages specifically designed for data analytics, making them popular choices among data analysts.
Additionally, relational databases and data management systems enable efficient data storage, retrieval, and query processing. These systems provide a structured approach to data management, ensuring data integrity and facilitating easy access to relevant information. With the help of database management systems, analysts can efficiently handle large volumes of data and perform complex queries to extract meaningful insights.
Various techniques are available for extracting insights from data. Regression analysis is a statistical technique used to model the relationship between a dependent variable and one or more independent variables. It helps analysts understand how changes in independent variables affect the dependent variable and make predictions based on the model.
Time series analysis is another powerful technique used in data analytics. It involves analyzing data collected over time to identify patterns, trends, and seasonal variations. This technique is widely used in forecasting future values based on historical data.
Machine learning algorithms have gained popularity in recent years for their ability to analyze large datasets and make predictions or decisions without explicit programming. These algorithms can automatically learn from data, identify patterns, and make accurate predictions or classifications. Machine learning techniques, such as clustering and classification, are widely used in various industries for tasks such as customer segmentation, fraud detection, and recommendation systems.
Visualization tools, such as Tableau and Power BI, allow for the creation of interactive dashboards and reports. These tools enable superintendents to communicate findings effectively and facilitate data-driven decision-making processes. Interactive dashboards provide a user-friendly interface for exploring data, allowing users to drill down into specific details and gain insights on the fly.
In conclusion, data analytics is a multidisciplinary field that combines various concepts, tools, and techniques to extract meaningful insights from data. By understanding the basic concepts and utilizing the right tools and techniques, superintendents can harness the power of data analytics to make informed decisions and drive organizational success.
Implementing Data Analytics in Sludge Management
Sludge management is a critical aspect of wastewater treatment plants, and incorporating data analytics can greatly enhance its efficiency and effectiveness. By leveraging data analytics techniques, organizations can gain valuable insights into their sludge management processes, leading to improved decision-making, cost savings, and environmental sustainability.
Steps to Incorporate Data Analytics
Integrating data analytics into sludge management requires a systematic approach. The following steps can guide you in successfully implementing data analytics within your organization:
- Define clear objectives: To begin, it is essential to identify specific goals that data analytics can help achieve. For instance, you may aim to improve process efficiency by optimizing sludge treatment or reduce maintenance costs by predicting equipment failures.
- Data collection and integration: Gathering relevant data is crucial for effective data analytics. This involves collecting data from various sources, such as sensors, databases, and external systems. By integrating data from different sources, you can create a comprehensive and reliable dataset that provides a holistic view of your sludge management processes.
- Data preprocessing: Before analysis, it is necessary to cleanse and transform the collected data to ensure its quality and compatibility. This step involves handling missing values, outliers, and inconsistencies, which can significantly impact the accuracy and reliability of the subsequent analysis.
- Exploratory data analysis: Conducting initial analysis is crucial to gain a deeper understanding of the characteristics of the data. Exploratory data analysis techniques, such as data visualization and statistical analysis, can help identify patterns, trends, and potential issues that may require further investigation.
- Model development: Selecting appropriate techniques and developing predictive or descriptive models is a key step in leveraging data analytics. These models should align with the objectives identified earlier and can include machine learning algorithms, statistical models, or optimization techniques.
- Evaluation and refinement: Assessing the accuracy and reliability of the developed models is essential to ensure their effectiveness. By evaluating the performance of the models against relevant metrics, you can identify areas for improvement and refine the models iteratively.
- Implementation and deployment: Integrating the developed models into the daily workflow of your organization is crucial for realizing the benefits of data analytics. This step involves training staff on using and interpreting the results generated by the models, as well as ensuring seamless integration with existing systems and processes.
- Continuous monitoring and improvement: Data analytics is an ongoing process, and regular monitoring is necessary to ensure the continued performance of the implemented analytics system. As new data and insights become available, it is important to update models and methodologies to adapt to changing conditions and optimize sludge management processes.
Overcoming Challenges in Implementation
Implementing data analytics in sludge management may present challenges, but with careful planning and execution, these obstacles can be overcome. Some common challenges include data quality issues, technical infrastructure limitations, and resistance to change.
Data quality issues: To address data quality issues, it is crucial to implement data governance practices that ensure data accuracy, consistency, and integrity. Regularly validating and cleansing the data can help maintain its reliability throughout the analysis process.
Technical infrastructure limitations: Overcoming technical infrastructure limitations requires investing in the necessary hardware, software, and networking capabilities to support data analytics initiatives. Collaborating with IT personnel is essential to ensure the availability of required resources and system compatibility.
Resistance to change: Tackling resistance to change involves emphasizing the benefits and value that data analytics can bring to the organization. Educating and training staff on the importance of data-driven decision-making and involving them in the implementation process can help foster a culture that promotes continuous improvement and encourages innovation.
By addressing these challenges and following a systematic approach, organizations can successfully implement data analytics in sludge management, leading to improved operational efficiency, cost savings, and sustainable wastewater treatment practices.
Analyzing and Interpreting Data for Decision Making
Techniques for Data Interpretation
Data interpretation is a crucial step in the data analytics process. Superintendents can utilize various techniques to derive meaningful insights from their data:
- Descriptive statistics: Summarize and describe the main characteristics of the data, such as means, medians, and standard deviations.
- Correlation analysis: Identify relationships and dependencies between variables to understand how they impact each other.
- Time series analysis: Analyze patterns and trends over time to detect seasonality, cyclicity, and long-term changes.
- Hypothesis testing: Statistically test assumptions and hypotheses about the data to make reliable conclusions.
Making Informed Decisions Based on Data
Ultimately, the purpose of data analytics in sludge management is to enable informed decision-making. By leveraging the insights gained from data analysis, superintendents can make decisions that are based on evidence and data-driven reasoning.
When making decisions based on data, consider the context, limitations, and uncertainties associated with the insights derived. Collaborate with other stakeholders, such as engineers, regulators, and finance personnel, to ensure a holistic decision-making process based on a multi-faceted understanding of the problem.
Ensuring Data Security and Compliance in Sludge Management
Understanding Data Privacy Regulations
Data security and privacy are of utmost importance in the era of digitalization. As a superintendent, you must adhere to data privacy regulations and protect sensitive information. Familiarize yourself with laws and regulations relevant to sludge management, such as the General Data Protection Regulation (GDPR) or local data protection laws. Implement appropriate measures to ensure data privacy and compliance, such as using encryption, access controls, and anonymization techniques when handling sensitive data.
Best Practices for Data Security
Implementing best practices for data security can help safeguard your organization's data and prevent unauthorized access. These practices include:
- Regularly updating software and systems to protect against known vulnerabilities.
- Using strong passwords and enabling multi-factor authentication.
- Limiting access to data based on job roles and responsibilities.
- Monitoring and logging access to sensitive data.
- Establishing backup and disaster recovery plans to ensure data resilience.
Future Trends in Data Analytics for Sludge Management
Predictive Analytics and AI in Sludge Management
Looking ahead, the adoption of predictive analytics and artificial intelligence (AI) is set to revolutionize the sludge management industry. Predictive analytics models can generate accurate forecasts, enabling superintendents to anticipate system behavior and address issues proactively.
AI technologies, such as machine learning algorithms, can automate data analysis processes, identify patterns, and make real-time decisions. These technologies can optimize system performance, reduce human error, and enhance overall efficiency.
The Impact of Big Data on Sludge Management
With the increasing availability of data from various sources, such as sensors, Internet of Things (IoT) devices, and advanced monitoring systems, the concept of big data is becoming more relevant in sludge management. Big data analytics can uncover hidden patterns and correlations, enabling superintendents to gain deeper insights into system behavior and performance. The analysis of large datasets combined with advanced analytical techniques can facilitate predictive maintenance, optimize resource allocation, and improve the decision-making processes of sludge management superintendents.
In conclusion, data analytics plays a crucial role in enabling superintendents to make informed decisions, optimize operational processes, and drive continuous improvement in the sludge management industry. By understanding the importance, fundamentals, and implementation of data analytics, as well as ensuring data security and compliance, superintendents can actively contribute to the advancement of the industry and achieve sustainable outcomes. Embrace the power of data analytics and unlock the potential for enhanced performance and cost savings in sludge management.