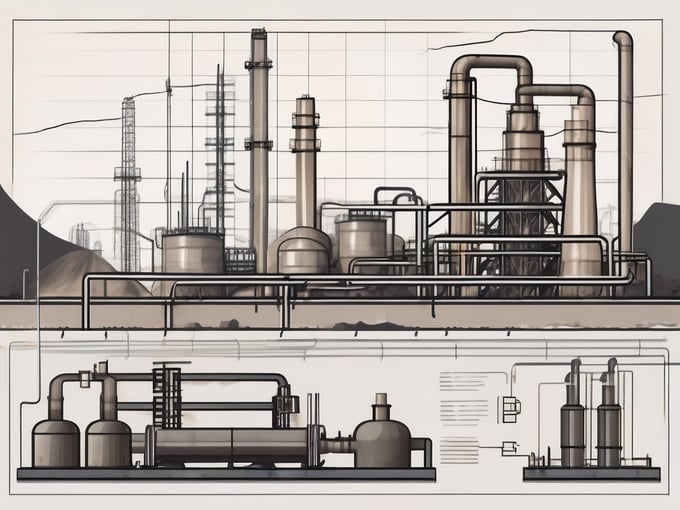
Important Trends in Data Analytics for Technicians in the Sludge Management Industry
In the sludge management industry, data analytics has emerged as a key tool for technicians seeking to improve their operations and make informed decisions. By harnessing the power of data, technicians can gain valuable insights that drive efficiency, enhance sustainability, and optimize sludge management processes. In this comprehensive guide, we will explore the importance of data analytics in sludge management, key concepts in data analytics, essential tools for technicians, implementation strategies, optimization techniques, and future trends in the field.
Understanding the Importance of Data Analytics in Sludge Management
Efficient sludge management is crucial for wastewater treatment facilities and environmental sustainability. As a technician in this industry, you have the responsibility of ensuring that sludge is properly treated, disposed of, or repurposed. To achieve these goals, you need accurate and reliable information, which can be obtained through data analytics.
Data analytics plays a pivotal role in transforming raw data gathered from various sources into actionable insights. By analyzing large datasets, technicians can identify patterns, trends, and correlations that help them make informed decisions in sludge management. This valuable information can guide operational strategies, resource allocation, and risk mitigation efforts.
One area where data analytics proves particularly useful is in optimizing the treatment process. By analyzing data on sludge composition, technicians can determine the most effective treatment methods and adjust the process parameters accordingly. For example, if the data reveals a high concentration of organic matter, the technicians can modify the treatment process to enhance the degradation of these compounds, leading to more efficient sludge treatment.
Furthermore, data analytics enables technicians to monitor the performance of sludge management systems in real-time. By continuously collecting and analyzing data from sensors and monitoring devices, technicians can detect any abnormalities or deviations from expected performance. This early detection allows for prompt intervention and prevents potential issues from escalating into major problems.
Benefits of Implementing Data Analytics in Your Operations
The implementation of data analytics in sludge management operations brings several benefits. By incorporating data-driven decision-making processes, technicians can optimize the treatment and disposal of sludge, reduce costs, and minimize environmental impact. Moreover, data analytics enables technicians to detect inefficiencies, predict maintenance needs, and identify potential issues before they become critical problems.
Another advantage of data analytics in sludge management is the ability to improve resource allocation. By analyzing historical data on sludge production and treatment, technicians can accurately forecast future sludge volumes and plan accordingly. This ensures that the necessary resources, such as storage capacity and treatment equipment, are available when needed, preventing bottlenecks and delays in the process.
In addition to operational benefits, data analytics also contributes to environmental sustainability. By analyzing data on sludge composition and environmental factors, technicians can identify opportunities for sludge repurposing or recycling. For example, if the data shows that the sludge contains high levels of nutrients, it can be used as a fertilizer in agricultural applications, reducing the need for synthetic fertilizers and promoting circular economy principles.
Furthermore, data analytics can support regulatory compliance efforts. By analyzing data on sludge quality and disposal practices, technicians can ensure that the facility meets all relevant environmental regulations and standards. This proactive approach not only avoids potential fines and penalties but also enhances the facility's reputation as a responsible and environmentally conscious organization.
In conclusion, data analytics plays a vital role in sludge management by providing technicians with valuable insights and information. By leveraging data analytics, technicians can optimize treatment processes, improve resource allocation, enhance environmental sustainability, and ensure regulatory compliance. Embracing data-driven decision-making processes is essential for wastewater treatment facilities to achieve efficient and effective sludge management.
Key Concepts in Data Analytics for Sludge Management
To effectively utilize data analytics, technicians need a solid understanding of key concepts and techniques used in this field. Here are some fundamental concepts:
Data analytics has revolutionized the way sludge management is approached. By harnessing the power of data, technicians can now make informed decisions, optimize resource allocation, and improve overall operational efficiency. Let's explore some key concepts in data analytics for sludge management in more detail:
Introduction to Data Mining Techniques
Data mining techniques involve the extraction of meaningful patterns and knowledge from large datasets. By applying these techniques to sludge management data, technicians can uncover previously unknown relationships and trends, leading to more efficient and effective decision-making.
One popular data mining technique used in sludge management is association rule mining. This technique helps identify patterns and correlations between various factors, such as sludge composition, treatment methods, and environmental conditions. By understanding these relationships, technicians can optimize treatment processes, reduce costs, and minimize environmental impact.
Another important data mining technique is clustering analysis. This technique groups similar sludge samples together based on their characteristics, allowing technicians to identify different sludge types and tailor treatment strategies accordingly. By categorizing sludge based on its properties, technicians can optimize treatment processes and ensure compliance with regulatory standards.
Predictive Analytics and Its Relevance
Predictive analytics utilizes historical data to predict future outcomes. In the context of sludge management, predictive analytics can help technicians anticipate sludge production patterns, maintenance requirements, and resource demands. This proactive approach enables technicians to plan ahead and optimize their operations accordingly.
One application of predictive analytics in sludge management is forecasting sludge production. By analyzing historical data on sludge production rates, technicians can develop models that predict future sludge volumes based on factors such as population growth, industrial activities, and weather patterns. This enables them to allocate resources, such as storage capacity and treatment equipment, more effectively.
Furthermore, predictive analytics can also be used to optimize maintenance schedules for sludge treatment facilities. By analyzing historical maintenance records and equipment performance data, technicians can identify patterns and predict when maintenance is likely to be required. This proactive approach helps prevent unexpected breakdowns, reduces downtime, and ensures the continuous operation of sludge treatment processes.
The Power of Real-Time Analytics
Real-time analytics involves the analysis of data as it is collected, allowing technicians to make instant decisions. By leveraging real-time analytics in sludge management, technicians can respond promptly to changes, optimize resource allocation, and prevent operational bottlenecks. Real-time analytics empowers technicians with timely insights, increasing operational efficiency.
In sludge management, real-time analytics can be applied to monitor key parameters such as sludge flow rates, pH levels, and temperature. By continuously analyzing these parameters in real-time, technicians can detect anomalies or deviations from normal operating conditions. This allows them to take immediate corrective actions, such as adjusting treatment processes or initiating maintenance procedures, to ensure optimal sludge treatment performance.
Furthermore, real-time analytics can also be used to optimize resource allocation in sludge management. By continuously monitoring resource usage, such as energy consumption, chemical dosing, and manpower, technicians can identify areas of inefficiency and implement corrective measures in real-time. This helps reduce costs, minimize waste, and improve overall operational sustainability.
As data analytics continues to evolve, its applications in sludge management are becoming increasingly sophisticated. By embracing these key concepts and techniques, technicians can unlock the full potential of data analytics and drive continuous improvement in sludge management practices.
Essential Data Analytics Tools for Technicians
Technicians can leverage a range of data analytics tools to effectively analyze and visualize sludge management data. Understanding these tools and selecting the right ones for your specific needs is crucial. Here are two essential tools:
Overview of Data Analytics Software
Data analytics software provides powerful features for data manipulation, analysis, and visualization. These tools allow technicians to perform complex calculations, generate reports, and create interactive dashboards. With the increasing volume and complexity of data in the field of sludge management, data analytics software plays a vital role in helping technicians make sense of the information at hand.
Tableau, one of the leading data analytics software, offers a user-friendly interface that allows technicians to easily connect to various data sources and create visually appealing dashboards. Its drag-and-drop functionality enables technicians to quickly analyze and explore data without the need for extensive coding knowledge. Power BI, another popular tool, provides seamless integration with Microsoft products and offers a wide range of data connectors, making it a versatile choice for technicians working in an environment heavily reliant on Microsoft technologies.
In addition to these well-established tools, the open-source programming language R is gaining popularity among technicians in the field of data analytics. R provides a vast array of statistical and graphical techniques, making it a powerful tool for analyzing and visualizing sludge management data. Its extensive library of packages allows technicians to access a wide range of functionalities, ensuring that they have the necessary tools to tackle any data-related challenges that may arise.
Selecting the Right Data Analytics Tools
When selecting data analytics tools, technicians should consider factors such as ease of use, compatibility with existing systems, scalability, and cost. It is important to choose tools that align with your specific goals and requirements. For instance, if your organization already uses Microsoft products extensively, Power BI may be the most suitable choice due to its seamless integration capabilities.
Scalability is another crucial factor to consider when selecting data analytics tools. As the volume of sludge management data grows over time, it is important to choose tools that can handle increasing data sizes without compromising performance. Some tools offer cloud-based solutions, allowing technicians to easily scale up their analytics capabilities as needed.
Cost is also a significant consideration. While some data analytics tools may come with a hefty price tag, there are also open-source options available that provide powerful functionalities at no cost. Technicians should carefully evaluate their budget and weigh the benefits of each tool before making a decision.
Additionally, staying updated with the latest advancements and emerging tools in the field ensures that you are utilizing the most efficient and effective solutions available. The field of data analytics is constantly evolving, with new tools and techniques being developed regularly. By staying informed and exploring new possibilities, technicians can stay ahead of the curve and continuously improve their data analytics capabilities.
Implementing Data Analytics in Sludge Management
Implementing data analytics in sludge management requires a structured approach. Here are the steps to incorporate data analytics into your operations:
Steps to Incorporate Data Analytics
1. Define Objectives: Clearly outline the goals and objectives you want to achieve through data analytics. This will guide your analysis and help you stay focused throughout the process.
When defining objectives, it is important to consider the specific challenges and pain points in your sludge management operations. For example, you may want to improve the efficiency of sludge dewatering or optimize the dosage of chemicals used in the treatment process. By setting clear objectives, you can ensure that your data analytics efforts are targeted and impactful.
2. Identify Data Sources: Determine the most relevant and valuable data sources for your objectives. This could include data from sensors, plant management systems, laboratory results, and historical records.
When identifying data sources, it is important to consider both internal and external sources. Internal sources may include data generated by your own sludge management processes, while external sources may include industry benchmarks or regulatory data. By considering a wide range of data sources, you can gain a comprehensive understanding of your sludge management operations.
3. Data Collection and Cleaning: Gather the identified data sources and ensure they are clean, accurate, and properly formatted for analysis. This may involve removing errors, duplicates, and outliers.
Data cleaning is a critical step in the data analytics process. It ensures that the data used for analysis is reliable and free from any inconsistencies. Data cleaning techniques may include data validation, data transformation, and outlier detection. By investing time and effort in data cleaning, you can improve the accuracy and reliability of your data analytics results.
4. Data Analysis: Apply appropriate data analytics techniques to extract meaningful insights. This can involve statistical analysis, data mining, visualization, and machine learning algorithms.
Data analysis techniques can vary depending on the objectives of your sludge management operations. For example, if your objective is to optimize sludge dewatering, you may use statistical analysis to identify the key factors affecting dewatering performance. On the other hand, if your objective is to predict sludge production, you may use machine learning algorithms to develop predictive models. By selecting the right data analysis techniques, you can uncover valuable insights and make data-driven decisions.
5. Interpretation and Action: Analyze the results obtained from your data analysis and translate them into actionable insights. Use these insights to drive decision-making processes and improve your sludge management operations.
Interpreting the results of data analysis is crucial for deriving actionable insights. It involves understanding the implications of the analysis and identifying opportunities for improvement. By translating data analytics results into actionable insights, you can drive meaningful change in your sludge management operations.
Overcoming Challenges in Data Analytics Implementation
Implementing data analytics in sludge management may present challenges, such as data quality issues, lack of analytical skills, and resistance to change. To overcome these challenges, it is crucial to invest in data governance practices, provide training and resources for technicians, and communicate the benefits of data analytics to stakeholders.
Data quality issues can arise due to various factors, such as data entry errors or sensor malfunctions. To address these issues, it is important to establish data governance practices, including data validation procedures and regular data audits. By ensuring data quality, you can have confidence in the accuracy and reliability of your data analytics results.
Lack of analytical skills can be a barrier to implementing data analytics in sludge management. To overcome this challenge, organizations can provide training and resources for technicians involved in data analytics. This can include workshops, online courses, or hiring data analytics experts to support the implementation process. By building analytical capabilities within your organization, you can effectively leverage data analytics for continuous improvement.
Resistance to change is another common challenge in implementing data analytics. To address this, it is important to communicate the benefits of data analytics to stakeholders, including operators, managers, and decision-makers. By highlighting the potential for cost savings, operational efficiency, and improved environmental performance, you can gain buy-in and support for your data analytics initiatives.
A collaborative approach involving all stakeholders can help overcome resistance and ensure successful implementation. By involving operators, technicians, and managers in the data analytics process, you can leverage their expertise and insights to drive meaningful change. Regular communication and feedback loops can also help to address any concerns or challenges that arise during the implementation process.
Optimizing Sludge Management Processes through Data Analytics
Data analytics can significantly enhance the efficiency and sustainability of sludge management processes. Here are two ways in which data analytics can be used to optimize operations:
Improving Efficiency with Data-Driven Decisions
Data analytics provides technicians with valuable insights to streamline operations. By analyzing historical data and real-time information, technicians can identify areas of improvement, reduce operational bottlenecks, and optimize resource allocation. This data-driven approach leads to increased operational efficiency, reducing costs and enhancing overall productivity.
Enhancing Sustainability through Data Insights
Data analytics enables technicians to identify environmentally friendly solutions and practices in sludge management. By analyzing data related to energy usage, carbon emissions, and waste disposal, technicians can make informed decisions that reduce the environmental impact of their operations. These insights can guide the implementation of sustainable practices, ultimately contributing to a greener and more sustainable sludge management industry.
Future Trends in Data Analytics for Sludge Management
Data analytics in the sludge management industry is constantly evolving. Here are two future trends to watch:
The Rise of AI and Machine Learning in Data Analytics
Artificial Intelligence (AI) and Machine Learning (ML) are poised to revolutionize data analytics in sludge management. AI-powered algorithms can analyze vast amounts of data, identify complex patterns, and provide highly accurate predictions. By leveraging AI and ML, technicians can automate routine tasks, improve data accuracy, and uncover deep insights that drive operational excellence.
The Impact of Big Data on Sludge Management
With the increasing volume of data generated in the sludge management industry, the utilization of big data solutions becomes essential. Big data technologies enable technicians to process and analyze massive datasets quickly, uncovering hidden insights and correlations. By harnessing the power of big data, technicians can make more informed decisions, optimize resource management, and improve overall operational efficiency.
As a technician in the sludge management industry, embracing data analytics is essential for achieving operational excellence, improving efficiency, and promoting environmental sustainability. By understanding the importance of data analytics, key concepts, essential tools, implementation strategies, optimization techniques, and future trends, you can take the necessary steps to leverage data analytics for success in your sludge management operations.